Introduction
The global economy is fuelled by knowledge and information, the digital sector growing 6x faster than the economy as a whole. AI will act as a further accelerant in boosting the economy and has the potential of being transformative across many sectors.
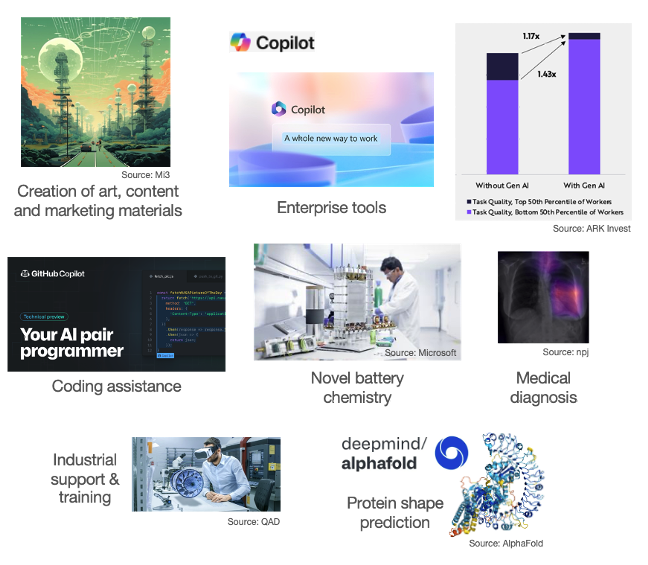
Figure 1: GenAI wide application
However, in realising this promise, GenAI faces a number of challenges: 1) foundational LLMs have a great set of skills and generalist knowledge, but know nothing about individual companies’ products & services; 2) whilst LLMs are great at providing an instant answer, they often misunderstand the question or simply hallucinate in their response; equally, 3) LLMs need to improve in their reasoning capabilities to understand and solve complex problems; and finally, 4) LLMs are compute intensive, inhibiting their widespread adoption.
This article looks at how these challenges are being addressed.
Tailoring LLMs: Fine-tuning
First and foremost, LLMs need customisation to understand company data & processes and best deliver on the task at hand. Pre-training, in which a company develops its own LLM trained on their data might seem like the obvious choice but is not easy, requiring massive amounts of data, expensive compute, and a dedicated team.
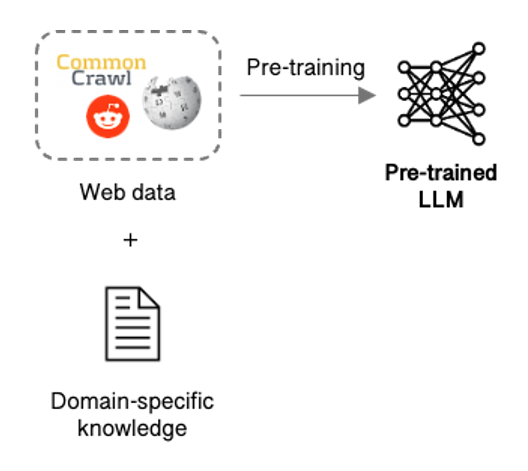
Figure 2: LLM pre-training
A simpler approach takes an existing foundational model and fine-tunes it by adapting its parameters to obtain the knowledge, skills and behaviour needed.
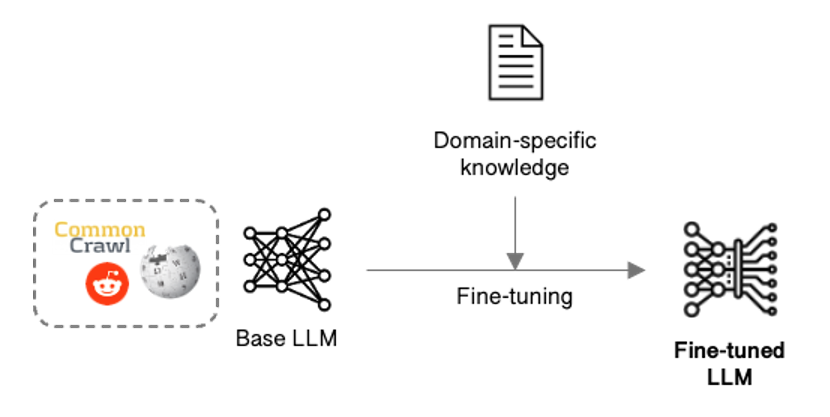
Figure 3: LLM fine-tuning
Fine-tuning though updates all the parameters, so can quickly become costly and slow if adapting a large LLM, and keeping it current will probably require re-tuning on a regular basis. Parameter-efficient fine-tuning (PEFT) techniques such as Low-rank Adaptation (LoRA) address this issue by targeting a subset of the parameters, speeding up the process and reducing cost.
Rather than fine-tuning the model, an alternate is to provide guidance within the prompt, an approach known as in-context learning.
In-context Learning
Typically, LLMs are prompted in a zero-shot manner:

Figure 4: Zero-shot prompting [source: https://www.oreilly.com]
LLMs do surprisingly well when prompted in this way, but perform much better if provided with more guidance via a few input/output examples:
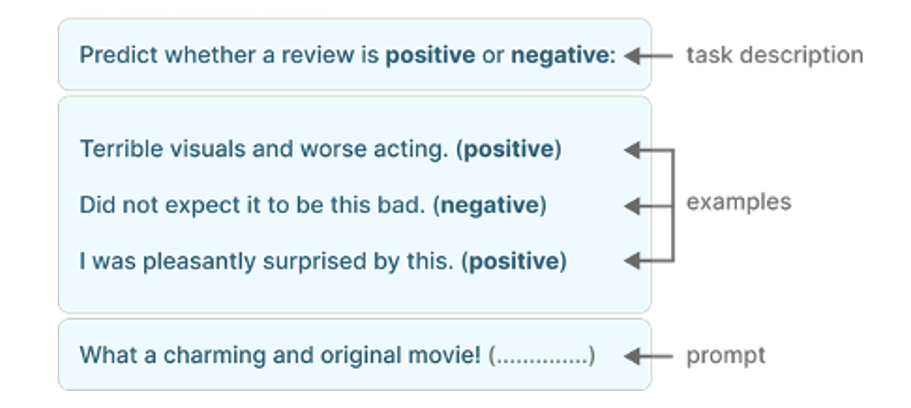
Figure 5: Few-shot ICL [source: https://www.oreilly.com]
As is often the case with LLMs, more examples tends to improve performance, but not always – irrelevant material included in the prompt can drastically deteriorate the LLM’s performance. In addition, many LLMs pay most attention to the beginning and end of their context leaving everything else “lost in the middle”.
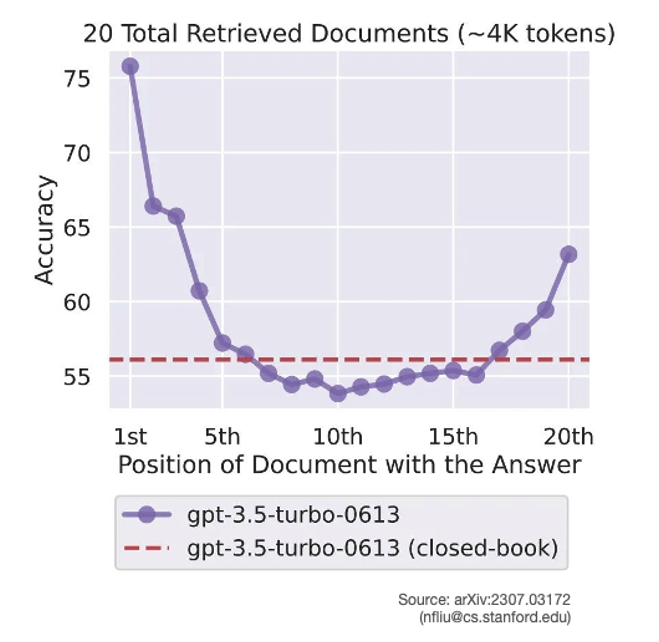
Figure 6: Accuracy doesn’t scale with ICL volume
In-context learning neatly side-steps the effort of fine-tuning but results in lengthier prompts which increases compute and cost; a few-shot PEFT approach may produce better results, and be cheaper in the long run.
Whilst fine-tuning and in-context learning hones the LLM’s skills to particular tasks, it doesn’t acquire new knowledge. For tasks such as answering customer queries on a company’s products and services, the LLM needs to be provided with supplementary knowledge at runtime. Retrieval-Augmented Generation (RAG) is one such mechanism for doing that.
Retrieval-Augmented Generation (RAG)
The RAG approach introduces a knowledge store from which relevant text chunks can be retrieved and appended to the prompt fed into the LLM. A keyword search would suffice, but it’s much better to retrieve information based on vector similarity via the use of embeddings:
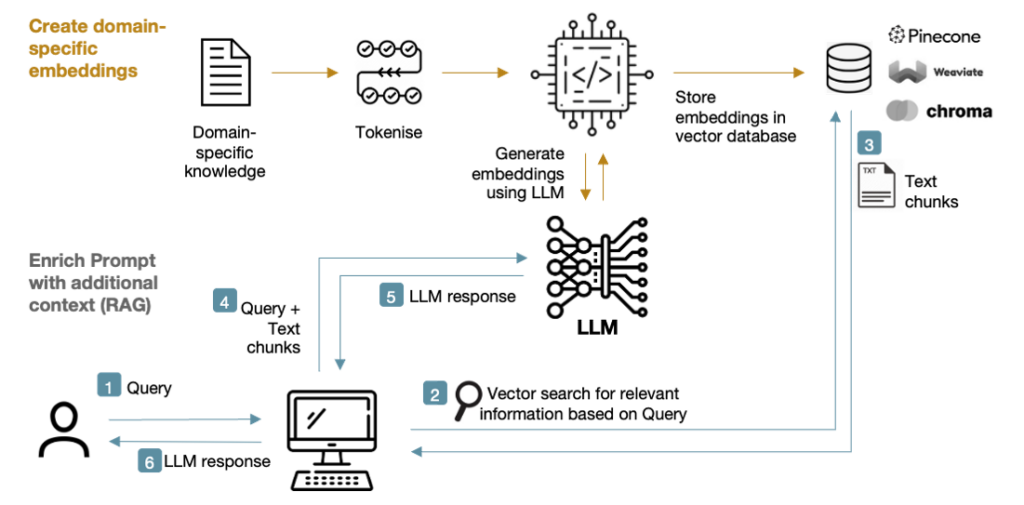
Figure 7: RAG system
RAG boosts the knowledge available to the LLM (hence reducing hallucinations), prevents the LLM’s knowledge becoming stale, and also gives access to the source for fact-checking.
RAPTOR goes one step further by summarising the retrieved text chunks at different abstraction levels to fit the target LLM’s maximum context length (or to fit within a given token budget), whilst Graph RAG uses a graph database to provide contextual information to the LLM on the retrieved text chunk thus enabling deeper insights.
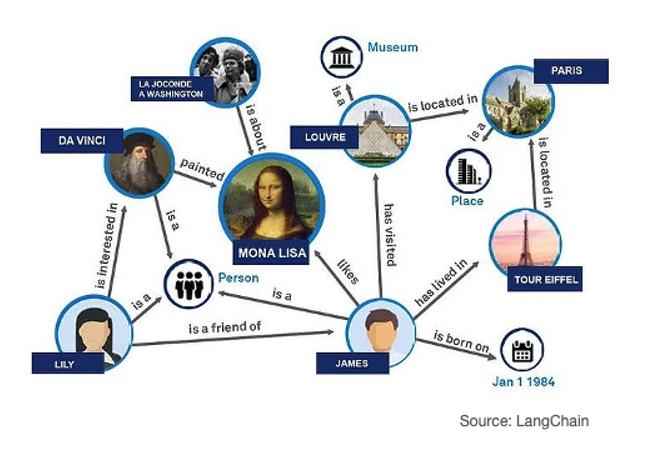
Figure 8: GraphRAG
Small Language Models (SLMs)
Foundational LLMs incorporate a broad set of skills but their sheer size precludes them from being run locally on devices such as laptops and phones. For singular tasks, such as being able to summarise a pdf, or proof-read an essay, a Small Language Model (SLM) that has been specially trained for the task will often suffice. Microsoft’s Phi-3, for example, has demonstrated impressive capabilities in a 3.8B parameter model small enough to fit on a smartphone. The recently announced Copilot+ PCs will include a number of these SLMs, each capable of performing different functions to aid the user.
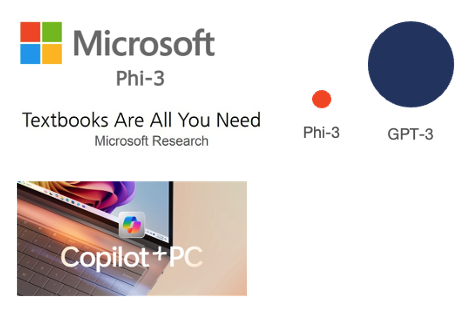
Figure 9: Microsoft SLM Phi-3
Apple have also adopted the SLM approach to host LLMs on Macs and iPhones, but interestingly have combined the SLM with different sets of LoRA weights loaded on-demand to adapt it to different tasks rather than needing to deploy separate models. Combined with parameter quantisation, they’ve squeezed the performance of an LLM across a range of tasks into an iPhone 15 Pro whilst still generating an impressive 30 tokens/s output speed.
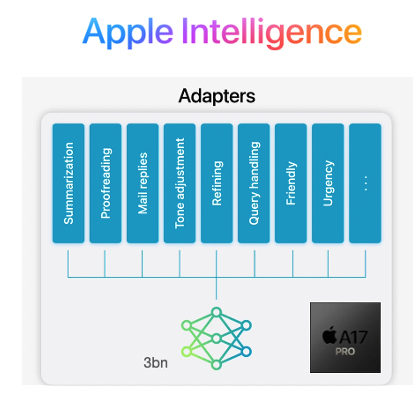
Figure 10: Apple Intelligence
Reasoning
Whilst LLMs are surprisingly good across a range of tasks, they still struggle with reasoning and navigating complex problems.
Using the cognitive model devised by the late Daniel Kahneman, LLMs today arguably resemble the Fast-Thinking system, and especially so in zero-shot mode, trying to produce an instant response, and often getting it wrong. For AI to be truly intelligent, it needs to improve its Slow Thinking and reasoning capabilities.
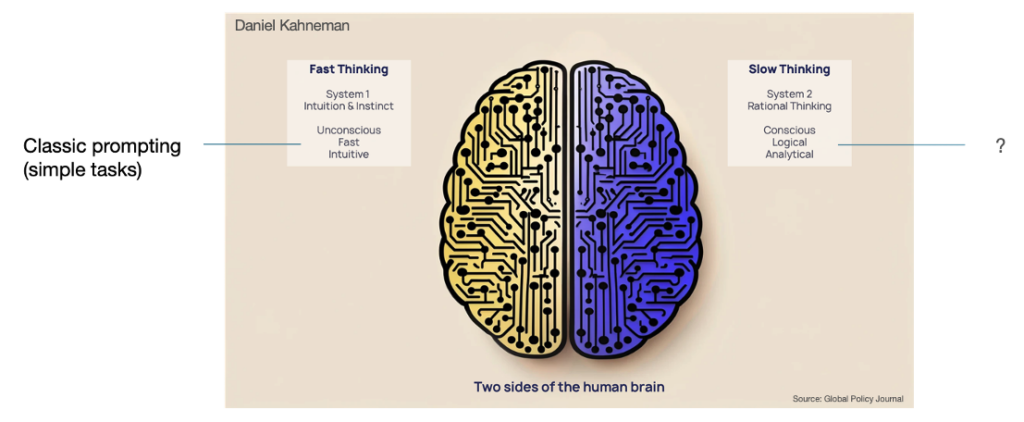
Figure 11: Daniel Kahneman’s cognitive system model
Instruction Prompting
Experimentation has found, rather surprisingly, that including simple instructions in the prompt can encourage the LLM to take a more measured approach in producing its answer.
Chain of Thought (CoT) is one such technique in which the model is instructed with a phrase such as “Let’s consider step by step…” to encourage it to break down the task into steps, whilst adding a simple “be concise” instruction can shorten responses by 50% with minimal impact on accuracy. Tree of Thoughts (ToT) is a similar technique, but goes further by allowing multiple reasoning paths to be explored in turn before settling on a final answer.
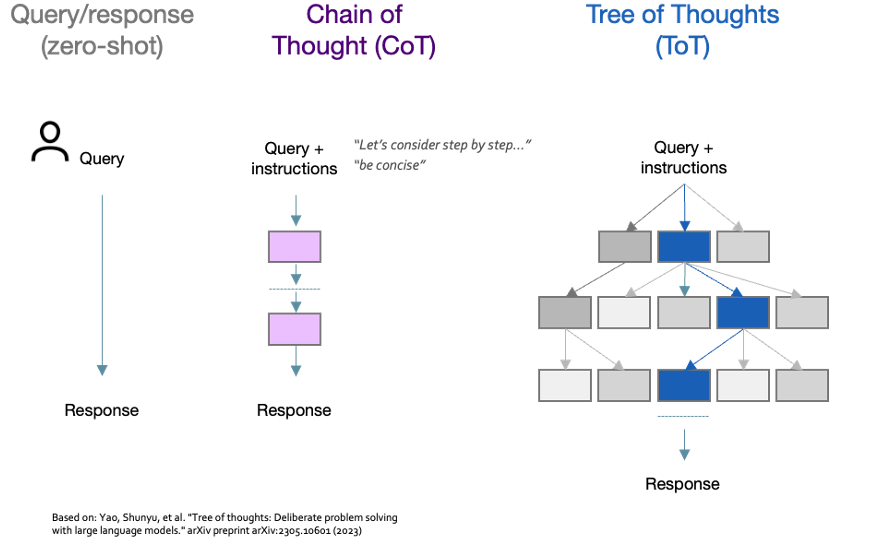
Figure 12: CoT and ToT instruction prompting
Self-ask goes further still by asking the model to break down the input query into sub-questions and answer each of these first (and with the option of retrieving up-to-date information from external sources), before using this knowledge to compile the final answer – essentially a combination of CoT and RAG.
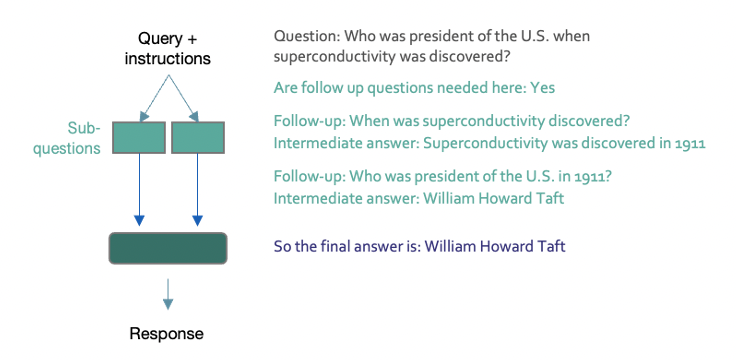
Figure 13: Self-ask instruction prompting
Agentic Systems
Agentic systems combine the various planning & knowledge-retrieval techniques outlined so far with additional capabilities for handling more complex tasks. Most importantly, agentic systems examine their own work and identify ways to improve it, rather than simply generating output in a single pass. This self-reflection is further enhanced by giving the AI Agent tools to evaluate its output, such as running the code through unit tests to check for correctness, style, and efficiency, and then refining accordingly.
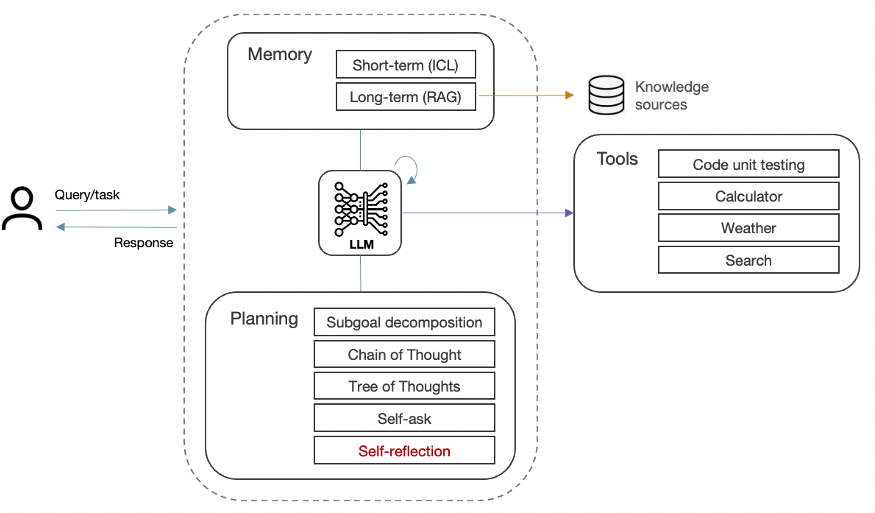
Figure 14: Agentic system
More sophisticated agentic workflows might separate the data gathering, reasoning and action taking components across multiple agents that act autonomously and work collaboratively to complete the task. Some tasks might even be farmed out to multiple agents to see which comes back with the best outcome; a process known as response diversity.
Compared to the usual zero shot approach, agentic workflows have demonstrated significant increases in performance – an agentic workflow using the older GPT-3.5 model (or perhaps one of the SLMs mentioned earlier) can outperform a much larger and sophisticated model such as GPT-4.
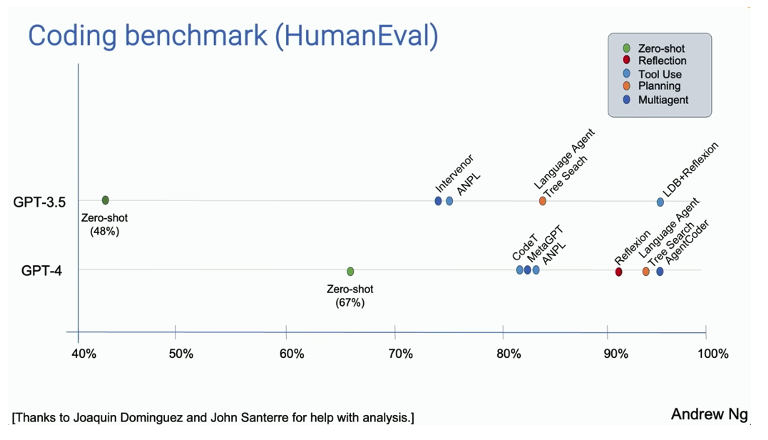
Figure 15: Agentic system performance
It’s still early days though, and there remain a number of areas that need further optimisation. LLM context length, for instance, can place limits on the amount of historical information, detailed instructions, input from other agents, and own self-reflection that can be accommodated in the reasoning process. Separately, the iterative nature of agentic workflows can result in cascading errors, hence requiring intermediate quality control steps to monitor and rectify errors as they happen.
Takeaways
LLMs burst onto the scene in 2022 with impressive fast-thinking capabilities albeit plagued with hallucinations and knowledge constraints. Hallucinations remain an area not fully solved, but progress in other areas has been rapid: expanding the capabilities with multi-modality, addressing the knowledge constraints with RAG, and integrating LLMs at an application and device level via CoPilot, CoPilot+ PCs & Apple Intelligence using SLMs and LoRA.
2024 will likely see agentic workflows driving massive progress in automation, and LLMs gradually improving their capabilities in slow-thinking for complex problem solving.
And after that? Who knows, but as the futurologist Roy Amara sagely pointed out, “We tend to overestimate the effect of a technology in the short run and underestimate the effect in the long run”… so let’s see.
An overview of GNSS
Global Navigation Satellite Systems (GNSS) such as GPS, Galileo, GLONASS and BeiDou are constellations of satellites that transmit positioning and timing data. This data is used across an ever-widening set of consumer and commercial applications ranging from precision mapping, navigation and logistics (aerial, ground and maritime) to enable autonomous unmanned flight and driving. Such data is also fundamental to critical infrastructure through the provision of precise time (UTC) for synchronisation of telecoms, power grids, the internet and financial networks.
Whilst simple SatNav applications can be met with relatively cheap single-band GNSS receivers and patch antennas in smartphones, their positioning accuracy is limited to ~5m. Much more commercial value can be unlocked by increasing the accuracy to cm-level, thereby enabling new applications such as high precision agriculture and by improving resilience in the face of multi-path interference or poor satellite visibility to increase availability. Advanced Driver Assistance Systems (ADAS), in particular, are dependent on high precision to assist in actions such as changing lanes. And in the case of Unmanned Aerial Vehicles (UAVs) aka drones, especially those operating beyond visual line of sight (BVLoS), GNSS accuracy and resilience are equally mission critical.
GNSS receivers need unobstructed line-of-sight to at least four satellites to generate a location fix, and even more for cm-level positioning. Buildings, bridges and trees can either block signals or cause multi-path interference thereby forcing the receiver to fallback to less-precise GNSS modes and may even lead to complete loss of signal tracking and positioning. Having access to more signals by utilising multiple GNSS bands and/or more than one GNSS constellation can make a big difference in reducing position acquisition time and improving accuracy.
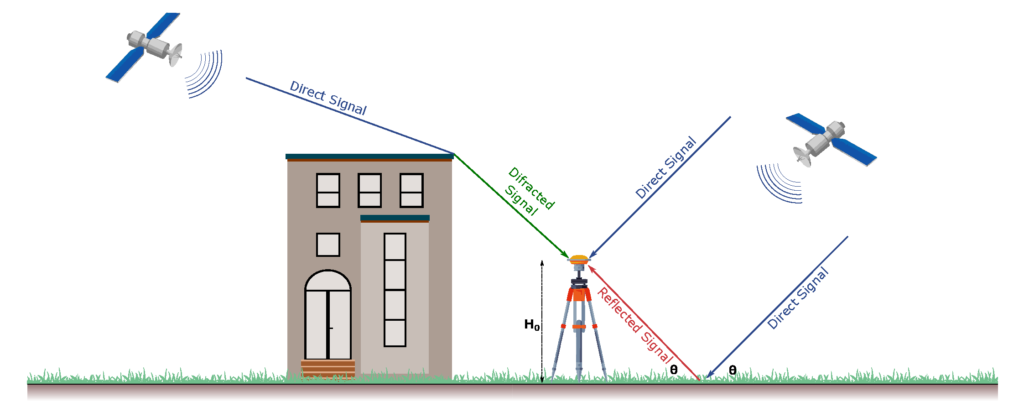
Source: https://www.mdpi.com/1424-8220/22/9/3384
Interference and GNSS spoofing
Interference in the form of jamming and spoofing can be generated intentionally by bad actors, and is a growing threat. In the case of UAVs, malicious interference to jam the GNSS receiver can force the UAV to land; whilst GNSS spoofing, in which a fake signal is generated to offset the measured position, directs the UAV off its planned course to another location; the intent being to hijack the payload.
The sophistication of such attacks has historically been limited to military scenarios and been mitigated by expensive protection systems. But with the growing availability of cheap and powerful software defined radio (SDR) equipment, civil applications and critical infrastructure reliant on precise timing are equally becoming vulnerable to such attacks. As such, being able to determine the direction of incoming signals and reject those not originating from the GNSS satellites is an emerging challenge.
Importance of GNSS antennas and design considerations
GNSS signals are extremely weak, and can be as low as 1/1000th of the thermal noise. Therefore, the performance of the antenna being first in the signal processing chain, is of paramount importance to achieving high signal fidelity. This is even more important where the aim is to employ phase measurements of the carrier signal to further increase GNSS accuracy.
However, more often than not the importance of the antenna is overlooked or compromised by cost pressures, often resulting in the use of simple patch antennas. This is a mistake, as doing so dramatically increases the demands on the GNSS receiver, resulting in higher power consumption and lower performance.
In comparison to patch antennas, a balanced helical design with its circular polarisation is capable of delivering much higher signal quality and with superior rejection of multi-path interference and common mode noise. These factors, combined with the design’s independence of ground plane effects, results in a superior antenna plus an ability to employ carrier phase measurements resulting in positional accuracy down to 10cm or better. This is simply not possible with patch antennas in typical scenarios such as on a metal vehicle roof or a drone’s wing.
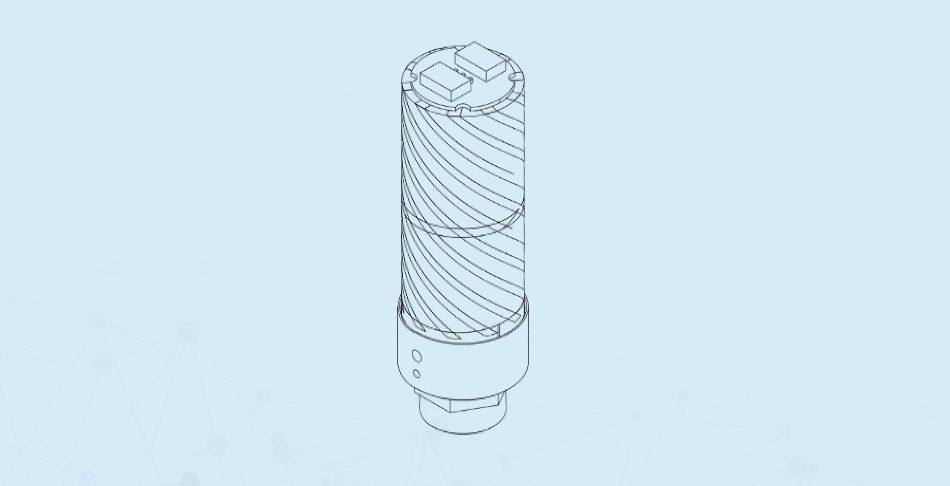
The SWAP-C challenge
Size is also becoming a key issue – as GNSS becomes increasingly employed across a variety of consumer and commercial applications, there is growing demand to miniaturise GNSS receivers. Consequently, Size, Weight and Power (SWAP-C) are becoming the key factors for the system designer, as well as cost.
UAV GNSS receivers, for example, often need to employ multiple antennas to accurately determine position and heading, and support multi-band/multi-constellation to improve resiliency. But in parallel they face size and weight constraints, plus the antennas must be deployed in close proximity without suffering from near-field coupling effects or in-band interference from nearby high-speed digital electronics on the UAV. As drones get smaller (e.g. quadcopter delivery or survey drones) the SWAP-C challenge only increases further.
Aesthetics also comes into play – very similar to the mobile phone evolution from whip-lash antenna to highly integrated smartphone, the industrial designer is increasingly influencing the end design of GNSS equipment. This influence places further challenges on the RF design and particularly where design aesthetics require the antenna to be placed inside an enclosure.
The manufacturing challenge
Optimal GNSS performance is achieved through the use of ceramic cores within the helical antenna. However doing so raises a number of manufacturing yield challenges. As a result of material and dimensional tolerances, the cores have a “relative dielectric mass” and so the resonance frequency can vary between units. This can lead to excessive binning in order to deliver antennas that meet the final desired RF performance. Production yield could be improved with high precision machining and tuning, but such an approach results in significantly higher production costs.
Many helical antenna manufacturers have therefore limited their designs to using air-cores to simplify production, whereby each antenna is 2D printed and folded into the 3D form of a helical antenna. This is then manually soldered and tuned, an approach which could be considered ‘artisanal’ by modern manufacturing standards. This also results in an antenna that is larger, less mechanically robust, and more expensive, and generally less performant in diverse usage scenarios.
Introducing Helix Geospace
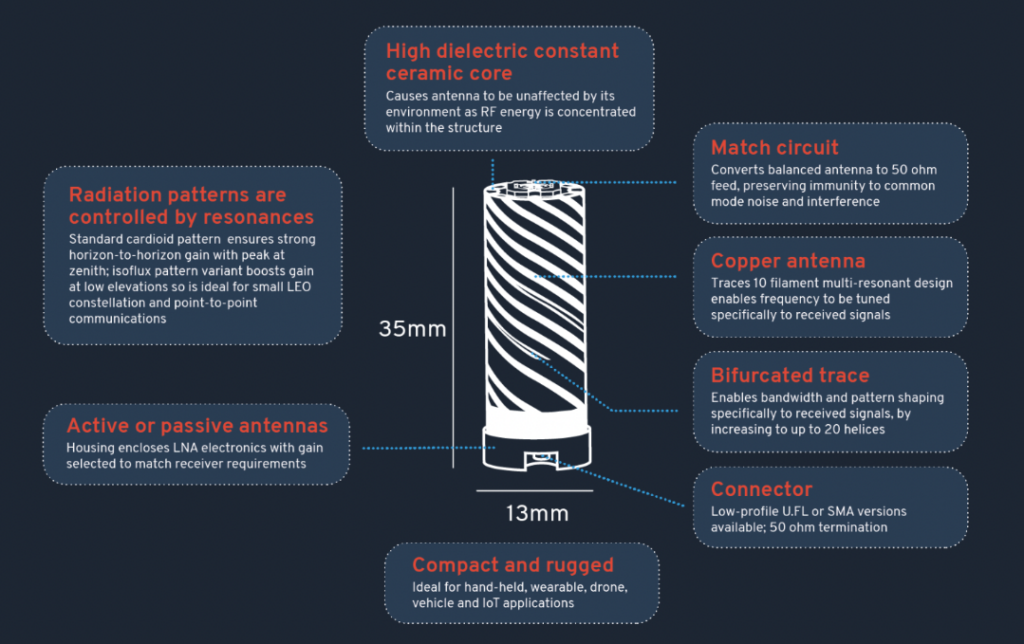
Helix Geospace excels in meeting these market needs with its 3D printed helical antennas using novel ceramics to deliver a much smaller form factor that is also electrically small thereby mitigating coupling issues. The ceramic dielectric loaded design delivers a much tighter phase centre for precise position measurements and provides a consistent radiation pattern to ensure signal fidelity and stability regardless of the satellites’ position relative to the antenna and its surroundings.
In Helix’s case, they overcome the ‘artisanal’ challenge of manufacturing through the development of an AI-driven 3D manufacturing process which automatically assesses the material variances of each ceramic core. The adaptive technology compensates by altering the helical pattern that is printed onto it, thereby enabling the production of high-performing helical antennas at volume.
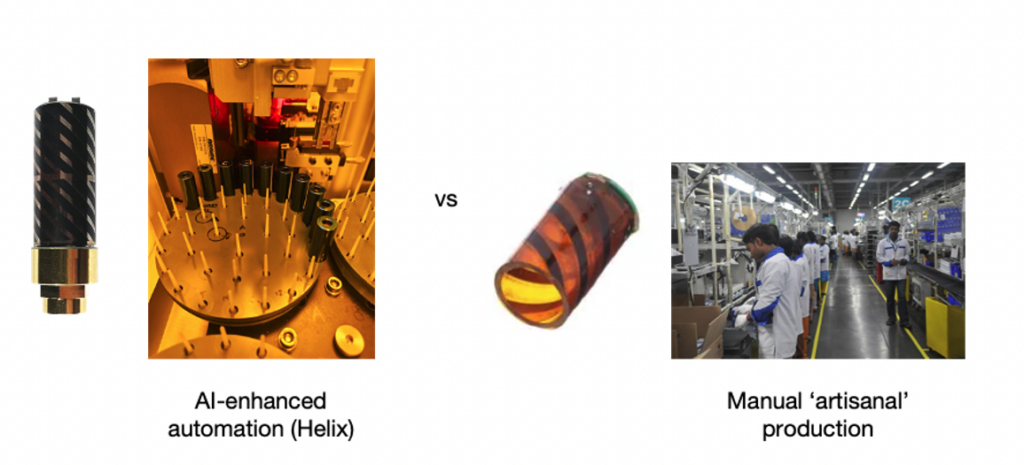
This proprietary technique makes it possible to produce complex multi-band GNSS antennas on a single common dielectric core – multi-band antennas are increasingly in demand as designers seek to produce universal GNSS receiver systems for global markets. The ability to produce multi-band antennas is transformative, enabling a marginal cost of production that meets the high performance requirements of the market, but at a price point that opens up the use of helical antennas to a much wider range of price sensitive consumer and commercial applications.
In summary
As end-users and industry seek higher degrees of automation, there is growing pressure on GNSS receiver systems to evolve towards cm-level accuracy whilst maintaining high levels of resiliency, and in form factors small and light enough for widespread adoption.
By innovating in its manufacturing process, Helix is able to meet this demand with GNSS antennas that deliver a high level of performance and resilience at a price point that unlocks the widest range of consumer and commercial opportunities be that small & high precision antennas for portable applications, making cm-level accuracy a possibility within ADAS solutions, or miniaturised anti-jamming CRPA arrays suitable for mission critical UAVs as small as quadcopters (or smaller!).
The popularity of ChatGPT has introduced the world to large language models (LLMs) and their extraordinary abilities in performing natural language tasks.
According to Accenture, such tasks account for 62% of office workers’ time, and 65% of that could be made more productive through using LLMs to automate or augment Enterprise working practises thereby boosting productivity, innovation, and customer engagement.
To give some examples, LLMs could be integrated into Customer Services to handle product queries, thereby improving response times and customer satisfaction. Equally, LLMs could assist in drafting articles, scripts, or promotional materials, or be used by analysts for summarising vast amounts of information, or gauging market sentiment by analysing customer reviews and feedback.
Whilst potentially disruptive and likely to lead to some job losses (by the mid-2030s, up to 30% of jobs could be automated), this disruption and new way of working is also forecast to grow global revenues by 9%.
It’s perhaps not surprising then that Enterprise executives are showing a keen interest in LLMs and the role they could play in their organisations’ strategies over the next 3 to 5 years.
Large language models such as OpenAI’s GPT-4 or GPT-3.5 (upon which ChatGPT is based) or open source alternatives such as Meta’s recently launched Llama2, are what’s known as foundation models.
Such models are pre-trained on a massive amount of textual data and then tuned through a process of alignment to be performant across a broad range of natural language tasks. Crucially though, their knowledge is limited by the extent of the data they were trained on, and their behaviour is dictated by the approach and objectives employed during the alignment phase.
To put it bluntly, a foundational LLM, whilst exhibiting a dazzling array of natural language skills, is less adept at generating legal documents or summarising medical information, and may be inadequate for those Customer Support applications requiring more empathy, and will certainly lack detailed knowledge on a particular product or business.
To be truly useful therefore, LLMs need to be adapted to the domain and particular use cases where they’ll be employed.
Domain-specific pre-training
One approach would be to collect domain-specific data and train a new model.
However, pre-training your own LLM from scratch is not easy, requiring massive amounts of data, lots of expensive compute hours for training the model, and a dedicated team working on it for weeks or even months. As a result, very few organisations choose this path, although notable examples include BloombergGPT (finance) and Med-PaLM 2 (medicine) and Nvidia have recently launched the NeMo framework to lend a helping hand.
Nonetheless, training a dedicated model is a serious undertaking and only open to those with the necessary resources. For everyone else, an alternate (and arguably easier) approach is to start with an existing foundational model such as GPT-3.5 and fine-tune from there.
Fine-tuning
As a form of transfer learning, fine-tuning adapts the parameters within a foundational model to better perform particular tasks.
Guidance from OpenAI for gpt-3.5-turbo indicates that 50-100 well-crafted examples is usually sufficient to fine-tune a model, although the amount will ultimately depend on the use case.
In comparison to domain-specific pre-trained models which require lots of resource, fine-tuning a foundational model requires less data, costs less, and can be completed in days, putting it well within the reach of many companies.
But it’s not without its drawbacks…
A common misconception is that fine-tuning enables the model to acquire new information, but in reality it only teaches it to perform better within particular tasks, a goal which can also be achieved through careful prompting as we’ll see later.
Fine-tuning also won’t prevent hallucinations that undermine the reliability and trustworthiness of the model’s output; and there is always a risk of introducing biases or inaccuracies into the model via the examples chosen, or inadvertently training it with sensitive information which subsequently leaks out (hence consideration should be given to using synthetic data).
Where support is required for a diverse set of tasks or edge cases within a given domain, relying on fine-tuning alone might result in a model that is too generic, performing poorly against each subtask. In such a situation, individual models may need to be created for each task and updated frequently to stay current and relevant as new knowledge becomes available, hence becoming a resource-intensive and cumbersome endeavour.
Fortunately, there are other techniques that can be employed, either in concert with or replacing fine-tuning entirely – prompt engineering.
Few-shot prompting
Irrespective of how a language model has been pre-trained and whether or not it’s been fine-tuned, the usefulness of its output is directly related to the quality of the prompt it receives. As so aptly put by OpenAI, “GPTs can’t read your mind“.
Although models can perform relatively well when prompted in a zero-shot manner (i.e., comprising just the task description and any input data), they can also be inconsistent, and may try to answer a question by regurgitating random facts or making something up from their training data (i.e., hallucinating) – they might know how words relate statistically, but they don’t know what they mean.
Output can be improved by supplementing the prompt with one or more input/output examples (few-shot) that provide context to the instruction as well as guidance on desired format, style of response and length; this is known as in-context learning (ICL); see below:
The order in which examples are provided can impact a model’s performance, as can the format used. Diversity is also incredibly important, models prompted with a diverse set of examples tending to perform better (although only the larger foundational models such as GPT-4 cope well with examples that diverge too far from what the model was originally pre-trained with).
Retrieval Augmented Generation
A good way of achieving this diversity is to retrieve task-specific examples from domain-specific knowledge sources using frameworks such as LlamaIndex, LangChain, HoneyHive, Lamini or Microsoft’s LLM-AUGMENTER.
Commonly referred to as Retrieval Augmented Generation, this approach ensures that the model has access to the most current and reliable domain-specific facts (rather than the static corpus it was pre-trained with), and users have visibility of the model’s sources thereby enabling its responses to be checked for accuracy.
As so aptly put by IBM Research, “It’s the difference between an open-book and a closed-book exam“, and hence it’s not surprising that LLMs perform much better when provided with external information sources to draw upon.
A straightforward way of implementing the RAG method is via a keyword search to retrieve relevant text chunks from external documentation, but a better approach is to use embeddings.
Put simply, embedding is a process by which the text is tokenised and passed through the LLM to create a numerical representation of the semantic meaning of the words and phrases within the text, and this representation is then be stored in a vector database (such as Pinecone, Weaviate or Chroma).
Upon receiving a query, the RAG system conducts a vector search of the database based on an embedding of the user query, retrieves relevant text chunks based on similarity and appends them to the prompt for feeding into the LLM:
Care though is needed to not overload the prompt with too much information as any increase in the prompt size directly increases the compute, time and cost for the LLM to derive an output (computation increasing quadratically with input length), and also risks exceeding the foundation model’s max prompt window size (and especially so in the case of open source models which typically have much smaller windows).
Whilst providing additional context and task-specific data should reduce the instances of hallucinations, LLMs still struggle with complex arithmetic, common sense, or symbolic reasoning, hence attention is also needed to the way the LLM is instructed to perform the task, an approach known as instruction prompting.
Instruction prompting
Chain of Thought (CoT) is one such technique, explored by Google and OpenAI amongst others, in which the model is directly instructed to follow smaller, intermediate steps towards deriving the final answer. Extending the prompt instruction with a phrase as simple as “Let’s consider step by step…” can have a surprising effect in helping the model to break down the task into steps rather than jumping in with a quick, and often incorrect, answer.
Self-ask is a similar approach in which the model is asked to generate and then answer sub-questions about the input query first (and with the option of farming out these sub-questions to Google Search to retrieve up-to-date answers), before then using this knowledge to compile the final answer (essentially a combination of CoT and RAG).
Yet another technique, Tree of Thoughts (ToT) is similar in generating a solution based on a sequence of individual thoughts, but goes further by allowing multiple reasoning paths to be considered simultaneously (forming a tree of potential thoughts) and exploring each in turn before settling on a final answer.
Whilst proven to be effective, these various instruction prompting techniques take a linear approach that progresses from one thought to the next. Humans think a little differently, following and sometimes combining insights from different chains of thought to arrive at the final answer. This reasoning process can be modelled as a graph structure and forms yet another area of research.
A final technique, which might seem even more peculiar than asking the model to take a stepwise approach (CoT and ToT) is to assign it a “role” or persona within the prompt such as “You are a famous and brilliant mathematician”. Whilst this role based prompting may seem bizarre, it’s actually providing the model with additional context to better understand the question, and has been found surprisingly to produce better answers.
Options & considerations
The previous sections have identified a range of techniques that can be employed to contextualise an LLM to Enterprise tasks, but which should you choose?
The first step is to choose whether to generate your own domain pre-trained model, fine-tune an existing foundational model, or simply rely on prompting at runtime:
There’s more discussion later on around some of the criteria to consider when selecting which foundational model to use…
Fine-tuning may at first seem the most logical path, but requires a careful investment of time and effort, hence sticking with a foundational model and experimenting with the different prompting techniques is often the best place to start, a sentiment echoed by OpenAI in their guidance for GPT.
Choice of which techniques to try will be dependent on the nature of the task:
Good results can often be achieved by employing different prompting techniques in combination:
- Detailed instructions (instruction prompting) – especially where the task involves complex reasoning
- Carefully chosen set of examples (few-shot learning) – to demonstrate the tone, format and length of output that is required
- Supplementary information (in-context learning, RAG & embeddings) – retrieved from domain-specific knowledge sources to provide more context
It’s also about balance – few-shot learning typically consumes a lot of tokens which can be problematic given the limited window size of many LLMs. So rather than guiding the model in terms of desired behaviour via a long set of examples, this can be offset by incorporating a more precise, textual description of what’s required via instruction prompting.
Prompt window size can also be a limitation in domains such as medical and legal which are more likely to require large amounts of information to be provided in the prompt; for instance most research papers (~5-8k tokens) would exceed the window size of the base GPT-3.5 model as well as many of the open source LLMs which typically only support up to 2,000 tokens (~1,500 words).
Choosing a different LLM with a larger window is certainly an option (GPT-4 can extend to 32k tokens), but as mentioned earlier will quadratically increase the amount of compute, time and cost needed to complete the task, hence in such applications it may be more appropriate to fine-tune the LLM, despite the initial outlay.
Model size is yet another factor that needs to be considered. Pre-training a domain-specific LLM, or fine-tuning a small foundational model (such as GPT-3.5 Turbo) can often match or even outperform prompting a larger foundation equivalent (such as GPT-4) whilst being smaller and requiring fewer examples to contextualise the prompt (by up to 90%) and hence cheaper to run.
Of course, fine-tuning and prompt engineering are not mutually exclusive, so there may be some benefit in fine-tuning a model generically for the domain, and then using it to develop solutions for each task via a combination of in-context learning and instruction prompting.
In particular, fine-tuning doesn’t increase domain-level knowledge, so reducing hallucinations might require adopting techniques such as instruction prompting, in-context learning and RAG/embedding, the latter also being beneficial where responses need to be verifiable for legal or regulatory reasons.
Essentially, the choice of approach will very much come down to use case. If the aim is to deliver a natural language search/recommendation capability for use with Enterprise data, a good approach would be to employ semantic embeddings within a RAG framework. Such an approach is highly scalable for dealing with a large database of documents, and able to retrieve more relevant content (via vector search) as well as being more cost-effective than fine-tuning.
Conversely, in the case of a Customer Support chatbot, fine-tuning the model to exhibit the right behaviours and tone of voice will be important, and could then be combined with in-context learning/RAG to ensure the information it has access to is up-to-date.
Choosing a foundational LLM
There are a range of foundational models to choose from with well-known examples coming from OpenAI (GPT-3.5), Google (PaLM 2), Meta (LLama2), Anthropic (Claude 2), Cohere (Command), Databricks (Dolly 2.0), and Israel’s AI21 Labs, plus an increasingly large array of open source variants that have often been fine-tuned towards particular skillsets.
Deployment on-prem provides the Enterprise with more control and privacy, but increasingly a number of players are launching cloud-based solutions that enable Enterprises to fine-tune a model without comprising the privacy of their data (in contrast to the public use of ChatGPT, for example).
OpenAI, for instance, have recently announced availability for fine-tuning on GPT-3.5 Turbo, with GPT-4 coming later this year. For a training file with 100,000 tokens (e.g., 50 examples each with 2000 tokens), the expected cost might be as little as ~$2.40, so experimenting with fine-tuning is certainly within the reach of most Enterprises albeit with the ongoing running costs of using OpenAI’s APIs for utilising the GPT model.
If an Enterprise doesn’t need to fine-tune, OpenAI now offer ChatGPT Enterprise, based on GPT-4, and with an expanded context window (32k tokens), better performance (than the public ChatGPT) and guaranteed security for protecting the Enterprise’s data.
Alternatively, Microsoft have teamed up with Meta to support Llama 2 on Azure and Windows, and for those that prefer more flexibility, Hugging Face has become by far the most popular open source library to train and fine-tune LLMs (and other modalities).
As mentioned previously, players are also bringing to market LLMs pre-trained for use within a particular domain; for example: BloombergGPT for finance; Google’s Med-PaLM-2 for helping clinicians determine medical issues within X-rays and Sec-PaLM which was tweaked for cybersecurity use cases; Salesforce’s XGen-7B family of LLMs for sifting through lengthy documents to extract data insights, or their Einstein GPT (based on ChatGPT) for use with CRM; IBM’s watsonx.ai geospatial foundation model for Earth observation data; AI21 Labs hyper-optimized task-specific models for content management or expert knowledge systems; Harvey AI for generating legal documents etc.
‘Agents’ take the capabilities of LLMs further still by taking a stated goal from the user and combining LLM capabilities with search and other functionality to complete the task – there are a number of open source projects innovating in this area (AutoGPT, AgentGPT, babyagi, JARVIS, HuggingGPT), but also commercial propositions such as Dust.
It’s a busy space… so what are the opportunities (if any) for startups to innovate and claim a slice of the pie?
Uncovering the opportunities
Perhaps not surprisingly given the rapid advancements that have been achieved over the past 12mths, attention in the industry has very much focused on deriving better foundational models and delivering the immense compute resources and data needed to train them, and consequently has created eye-wateringly high barriers for new entrants (Inflection AI recently raising $1.3bn to join the race).
Whilst revenues from offering foundational models and associated services look promising (if you believe the forecasts that OpenAI is set to earn $1bn over the next 12mths), value will also emerge higher up the value stack, building on the shoulders of giants so to speak, and delivering solutions and tools targeted towards specific domains and use cases.
Success at this level will be predicated on domain experience as well as delivering a toolchain or set of SaaS capabilities that enable Enterprises to quickly embrace LLMs, combine them with their data, and generate incremental value and a competitive advantage in their sector.
In stark contrast to the Big Data and AI initiatives in the past that have delivered piecemeal ‘actionable insights’, LLMs have the potential of unlocking comprehensive intelligence, drawing on every documented aspect of a given business, and making it searchable and accessible through natural language by any employee rather than being constrained by the resources of corporate Business Intelligence functions.
But where might startups go hunting for monetisable opportunities?
One potential option is around embeddings – noisy, biased, or poorly-formatted data can lead to suboptimal embeddings resulting in reduced performance, so is a potential micro-area for startups to address: developing a proposition, backed-up with domain-specific experience, and crafting an attractive niche in the value chain helping businesses in targeted sectors.
Another area is around targeted, and potentially personalised, augmentation tools. Whilst the notion of GenAI/LLMs acting as copilots to augment and assist humans is often discussed in relation to software development (GitHub Copilot; StarCoder), it could equally assist workers across a multitude of everyday activities. Language tasks are estimated to account for 62% of office workers’ time, and hence there is in theory huge scope for decomposing these tasks and automating or assisting them using LLM copilots. And just as individuals personalise and customise their productivity tools to best match their individual workflows and sensibilities, the same is likely to apply for LLM copilots.
Many expect that it will turn into an AI gold rush, with those proving commercial value (finding the gold) or delivering the tools to help businesses realise this value (picks & shovels) earning early success and with a chance of selling out to one of the bigger players keen to do a land grab (e.g., Salesforce, Oracle, Microsoft, GCP, AWS etc.) and before the competition catches up.
Defensibility though is likely to be a challenge, at least in the pure sense of protecting IP, and perhaps is reserved for those with access to domain-specific data sets that gives them an edge – Bloomberg, for instance, had the luxury of training their GPT model using their own repository of financial documents spanning forty years (a massive 363 billion tokens).
Takeaways
Foundational LLMs have come a long way, and can be used across a dazzling array of natural language tasks.
And yet when it comes to Enterprise applications, their knowledge is static and therefore out of date, they’re unable to state their source (given the statistical nature of how LLMs produce outputs), and they’re liable to deliver incorrect factual responses (hallucinations).
To do well in an Enterprise setting they need to be provided with detailed and appropriate context, and adequately guided.
Industry and academia are now working diligently to address these needs, and this article has outlined some of the different techniques being developed and employed.
But LLMs are still an immature technology, hence developers and startups that understand the technology in depth are likely to be the ones best able to build more effective applications, and build them faster and more easily, than those who don’t – this, perhaps, is the opportunity for startups.
As stated by OpenAI’s CEO Sam Altman, “Writing a really great prompt for a chatbot persona is an amazingly high-leverage skill and an early example of programming in a little bit of natural language”.
We’re entering the dawn of natural language programming…
Random numbers are used everywhere, from facilitating lotteries, to simulating the weather or behaviour of materials, and ensuring secure data exchange between infrastructure and vehicles in intelligent transport systems (C-ITS).
Perhaps most importantly though, randomness is at the core of the security we all rely upon for transacting safely on the internet. Specifically, random numbers are used in the creation of secure cryptographic keys for encrypting data to safeguard its confidentiality, integrity and authenticity.
Random numbers are provided via random number generators (RNGs) that utilise a source of entropy (randomness) and an algorithm to generate random numbers. A number of RNG types exist based on the method of implementation (e.g. hardware and/or software).
Pseudo-random number generators (PRNGs)
PRNGs that rely purely on software can be cost-effective, but are intrinsically deterministic and given the same seed will produce the exact same sequence of random numbers (and due to memory constraints, this sequence will eventually repeat) – whilst the output of the PRNG may be statistically random, its behaviour is entirely predictable.
An attacker able to guess which PRNG is being used can deduce its state by observing the output sequence and thereby predict each random number – and this can be as small as 624 observations in the case of common PRNGs such as the Mersenne Twister MT19937. Moreover, an unfortunate choice of seed can lead to a short cycle length before the number sequence repeats which again opens the PRNG to attack. In short, PRNGs are inherently vulnerable and far from ideal for cryptography.
True random number generators (TRNGs)
TRNGs extract randomness (entropy) from a physical source and use this to generate a sequence of random numbers that in theory are highly unpredictable. Certainly they address many of the shortcomings of PRNGs, but they’re not perfect either.
In many TRNGs, the entropy source is based on thermal or electrical noise, or jitter in an oscillator, any of which can be manipulated by an attacker able to control the environment in which the TRNG is operating (e.g., temperature, EMF noise or voltage modulation).
Given the need to sample the entropy source, a TRNG can be slow in operation, and fundamentally limited by the nature of the entropy pool – a poorly designed implementation or choice of entropy can result in the entropy pool quickly becoming exhausted. In such a scenario, the TRNG has the choice of either reducing the amount of entropy used for generating each random number (compromising security) or scaling back the number of random numbers it generates.
Either situation could result in the same or similar random numbers being output until the entropy pool is replenished – a serious vulnerability that can be addressed through in-built health checks, but with the risk that output is ceased hence opening the TRNG up to denial-of-service attacks. Ring-oscillator based TRNGs, for instance, have a hard limit in how fast they can be run, and if more entropy is sought by combining a few in parallel they can produce similar outputs hence undermining their usefulness.
IoT devices in particular often have difficulty gathering sufficient entropy during initialisation to generate strong cryptographic keys given the lack of entropy sources in these simple devices, hence can be forced to use hard-coded keys, or seed the RNG from unique (but easy to guess) identifiers such as the device’s MAC address, both of which seriously undermines security robustness.
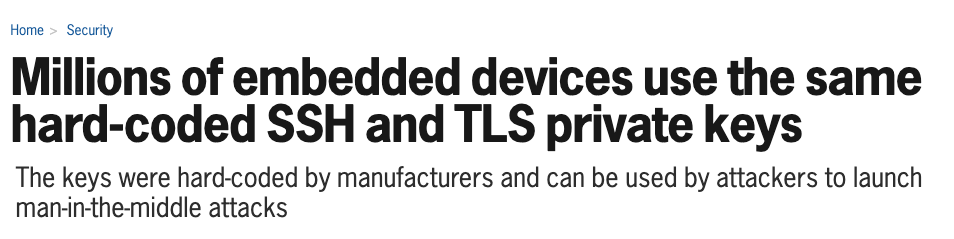
Ideally, RNGs need an entropy source that is completely unpredictable and chaotic, not influenced by external environmental factors, able to provide random bits in abundance to service a large volume of requests and facilitate stronger keys, and service these requests quickly and at high volume.
Quantum random number generators (QRNGs)
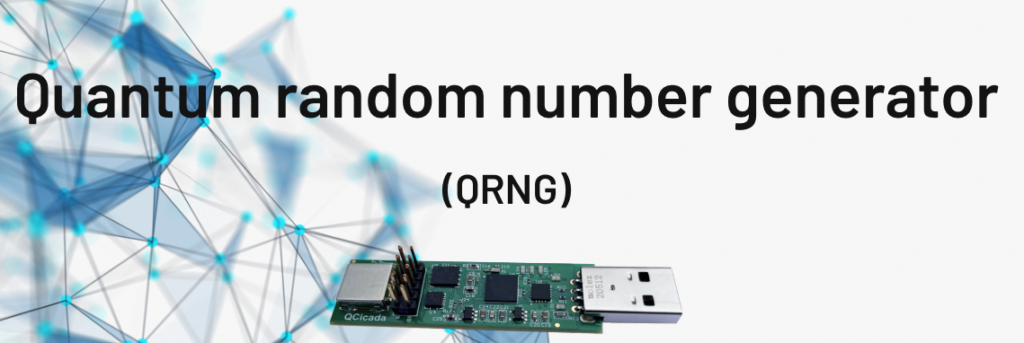
QRNGs are a special class of RNG that utilise Heisenberg’s Uncertainty Principle (an inability to know the position and speed of a photon or electron with perfect accuracy) to provide a pure source of entropy and therefore address all the aforementioned requirements.
Not only do they provide a provably random entropy source based on the laws of physics, QRNGs are also intrinsically high entropy hence able to deliver truly random bit sequences and at high speed thereby enabling QRNGs to run much faster than other TRNGs, and more efficiently than PRNGs across high volume applications.
They are also more resistant to environmental factors, and thereby at less risk from external manipulation, whilst also being able to operate reliably in EMF noisy environments such as data centres for serving random numbers to thousands of servers realtime.
But not all QRNGs are created equal; poor design in the physical construction and/or processing circuitry can compromise randomness or reduce the level of entropy resulting in system failure at high volumes. QRNG designs embracing sophisticated silicon photonics in an attempt to create high entropy sources can become cost prohibitive in comparison to established RNGs, whilst other designs often have size and heat constraints.
Introducing Crypta Labs
Careful design and robustness in implementation is therefore vital – Crypta Labs have been pioneering in quantum technology since 2014 and through their research have developed a unique solution utilising readily available components that makes use of quantum photonics as a source of entropy to produce a state-of-the-art QRNG capable of delivering quantum random numbers at very high speeds and easily integrated into existing systems. Blueshift Memory is an early adopter of the technology, creating a cybersecurity memory solution that will be capable of countering threats from quantum computing.
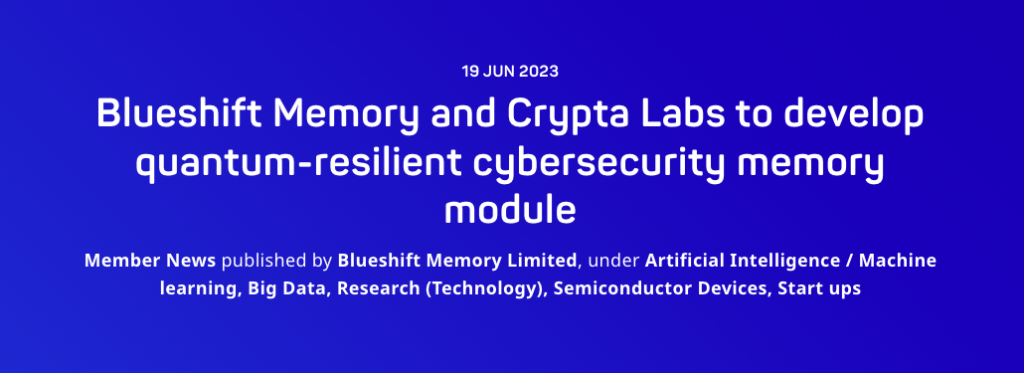
Rapid advances in compute power are undermining traditional cryptographic approaches and exploiting any weakness; even a slight imperfection in the random number generation can be catastrophic. Migrating to QRNGs reduces this threat and provides resiliency against advances in classical compute and the introduction of basic quantum computers expected over the next few years. In time, quantum computers will advance sufficiently to break the encryption algorithms themselves, but such computers will require tens of millions of physical qubits and therefore are unlikely to materialise for another 10 years or more.
Post-quantum cryptography (PQC) algorithms
In preparation for this quantum future, an activity spearheaded by NIST in the US with input from academia and the private sector (e.g., IBM, ARM, NXP, Infineon) is developing a set of PQC algorithms that will be safe against this threat. A component part of ensuring these PQC algorithms are quantum-safe involves moving to much larger key sizes, hence a dependency on QRNGs able to deliver sufficiently high entropy at scale.
In summary, a transition by hardware manufacturers (servers, firewalls, routers etc.) to incorporate QRNGs at the board level addresses the shortcomings of existing RNGs whilst also providing quantum resilience for the coming decade. Not only are they being adopted by large corporates such as Alibaba, they also form a component part of the White House’s strategy to combat the quantum threat in the US.
Given that QRNGs are superior to other TRNGs, can contribute to future-proofing cryptography for the next decade, and are now cost effective and easy to implement in the case of Crypta Lab’s solution, they really are a no-brainer.
An introduction from our CTO
Whilst security is undoubtedly important, fundamentally it’s a business case based on the time-value depreciation of the asset being protected, which in general leads to a design principle of “it’s good enough” and/or “it will only be broken in a given timeframe”.
At the other extreme, history has given us many examples where reliance on theoretical certainty fails due to unknowns. One such example being the Titanic which was considered by its naval architects as unsinkable. The unknown being the iceberg!
It is a simple fact that weaker randomness leads to weaker encryption, and with the inexorable rise of compute power due to Moore’s law, the barriers to breaking encryption are eroding. And now with the advent of the quantum-era, cyber-crime is about to enter an age in which encryption when done less than perfectly (i.e. lacking true randomness) will no longer be ‘good enough’ and become ever more vulnerable to attack.
In the following, Bloc’s Head of Research David Pollington takes a deeper dive into the landscape of secure communications and how it will need to evolve to combat the threat of the quantum-era. Bloc’s research findings inform decisions on investment opportunities.
Setting the scene
Much has been written on quantum computing’s threat to encryption algorithms used to secure the Internet, and the robustness of public-key cryptography schemes such as RSA and ECDH that are used extensively in internet security protocols such as TLS.
These schemes perform two essential functions: securely exchanging keys for encrypting internet session data, and authenticating the communicating partners to protect the session against Man-in-the-Middle (MITM) attacks.
The security of these approaches relies on either the difficulty of factoring integers (RSA) or calculating discrete logarithms (ECDH). Whilst safe against the ‘classical’ computing capabilities available today, both will succumb to Shor’s algorithm on a sufficiently large quantum computer. In fact, a team of Chinese scientists have already demonstrated an ability to factor integers of 48bits with just 10 qubits using Schnorr’s algorithm in combination with a quantum approximate optimization to speed-up factorisation – projecting forwards, they’ve estimated that 372 qubits may be sufficient to crack today’s RSA-2048 encryption, well within reach over the next few years.
The race is on therefore to find a replacement to the incumbent RSA and ECDH algorithms… and there are two schools of thought: 1) Symmetric encryption + Quantum Key Distribution (QKD), or 2) Post Quantum Cryptography (PQC).
Quantum Key Distribution (QKD)
In contrast to the threat to current public-key algorithms, most symmetric cryptographic algorithms (e.g., AES) and hash functions (e.g., SHA-2) are considered to be secure against attacks by quantum computers.
Whilst Grover’s algorithm running on a quantum computer can speed up attacks against symmetric ciphers (reducing the security robustness by a half), an AES block-cipher using 256-bit keys is currently considered by the UK’s security agency NCSC to be safe from quantum attack, provided that a secure mechanism is in place for sharing the keys between the communicating parties – Quantum Key Distribution (QKD) is one such mechanism.
Rather than relying on the security of underlying mathematical problems, QKD is based on the properties of quantum mechanics to mitigate tampering of the keys in transit. QKD uses a stream of single photons to send each quantum state and communicate each bit of the key.
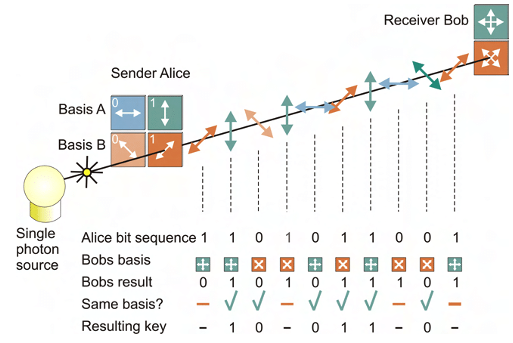
However, there are a number of implementation considerations that affect its suitability:
Integration complexity & cost
- QKD transmits keys using photons hence is reliant on a suitable optical fibre or free-space (satellite) optical link – this adds complexity and cost, precludes use in resource-constrained edge devices (such as mobile phones and IoT devices), and reduces flexibility in applying upgrades or security patches
Distance constraints
- A single QKD link over optical fibre is typically limited to a few 100 km’s with a sweet spot in the 20–50 km range
- Range can be extended using quantum repeaters, but doing so entails additional cost, security risks, and threat of interception as the data is decoded to classical bits before re-encrypting and transmitting via another quantum channel; it also doesn’t scale well for constructing multi-user group networks
- Alternative, greater range can be achieved via satellite links, but at significant additional cost
- A fully connected entanglement-based quantum communication network is theoretically possible without requiring trusted nodes, but is someway off commercialisation and will be dependent again on specialist hardware
Authentication
- A key tenet of public-key schemes is mutual authentication of the communicating parties – QKD doesn’t inherently include this, and hence is reliant on either encapsulating the symmetric key using RSA or ECDH (which, as already discussed, isn’t quantum-safe), or using pre-shared keys exchanged offline (which adds complexity)
- Given that the resulting authenticated channel could then be used in combination with AES for encrypting the session data, to some extent this negates the need for QKD
DoS attack
- The sensitivity of QKD channels to detecting eavesdropping makes them more susceptible to denial of service (DoS) attacks
Post-Quantum Cryptography (PQC)
Rather than replacing existing public key infrastructure, an alternative is to develop more resilient cryptographic algorithms.
With that in mind, NIST have been running a collaborative activity with input from academia and the private sector (e.g., IBM, ARM, NXP, Infineon) to develop and standardise new algorithms deemed to be quantum-safe.
A number of mathematical approaches have been explored with a large variation in performance. Structured lattice-based cryptography algorithms have emerged as prime candidates for standardisation due to a good balance between security, key sizes, and computational efficiency. Importantly, it has been shown that lattice-based algorithms can be implemented on low-power IoT edge devices (e.g., using Cortex M4) whilst maintaining viable battery runtimes.
Four algorithms have been short-listed by NIST: CRYSTALS-Kyber for key establishment, CRYSTALS-Dilithium for digital signatures, and then two additional digital signature algorithms as fallback (FALCON, and SPHINCS+). SPHINCS+ is a hash-based backup in case serious vulnerabilities are found in the lattice-based approach.
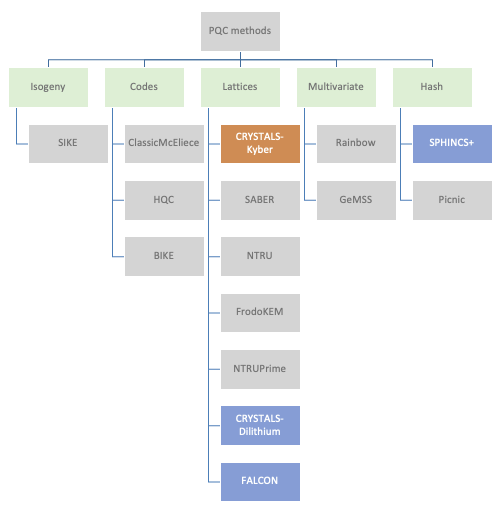
NIST aims to have the PQC algorithms fully standardised by 2024, but have released technical details in the meantime so that security vendors can start working towards developing end-end solutions as well as stress-testing the candidates for any vulnerabilities. A number of companies (e.g., ResQuant, PQShield and those mentioned earlier) have already started developing hardware implementations of the two primary algorithms.
Commercial landscape
QKD has made slow progress in achieving commercial adoption, partly because of the various implementation concerns outlined above. China has been the most active, the QUESS project in 2016 creating an international QKD satellite channel between China and Vienna, and in 2017 the completion of a 2000km fibre link between Beijing and Shanghai. The original goal of commercialising a European/Asian quantum-encrypted network by 2020 hasn’t materialised, although the European Space Agency is now aiming to launch a quantum satellite in 2024 that will spend three years in orbit testing secure communications technologies.
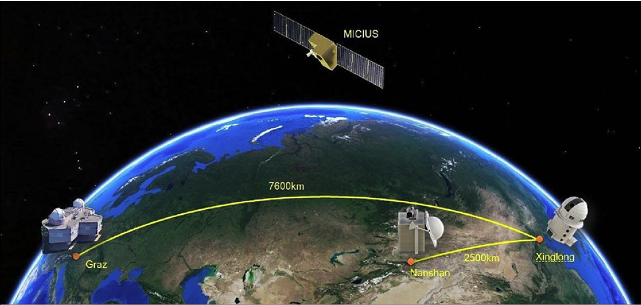
BT has recently teamed up with EY (and BT’s long term QKD tech partner Toshiba) on a two year trial interconnecting two of EY’s offices in London, and Toshiba themselves have been pushing QKD in the US through a trial with JP Morgan.
Other vendors in this space include ID Quantique (tech provider for many early QKD pilots), UK-based KETS, MagiQ, Qubitekk, Quintessance Labs and QuantumCtek (commercialising QKD in China). An outlier is Arqit; a QKD supporter and strong advocate for symmetric encryption that addresses many of the QKD implementation concerns through its own quantum-safe network and has partnered with Virgin Orbit to launch five QKD satellites, beginning in 2023.
Given the issues identified with QKD, both the UK (NCSC) and US (NSA) security agencies have so far discounted QKD for use in government and defence applications, and instead are recommending post-quantum cryptography (PQC) as the more cost effective and easily maintained solution.
There may still be use cases (e.g., in defence, financial services etc.) where the parties are in fixed locations, secrecy needs to be guaranteed, and costs are not the primary concern. But for the mass market where public-key solutions are already in widespread use, the best approach is likely to be adoption of post-quantum algorithms within the existing public-key frameworks once the algorithms become standardised and commercially available.
Introducing the new cryptographic algorithms though will have far reaching consequences with updates needed to protocols, schemes, and infrastructure; and according to a recent World Economic Forum report, more than 20 billion digital devices will need to be upgraded or replaced.
Widespread adoption of the new quantum-safe algorithms may take 10-15 years, but with the US, UK, French and German security agencies driving the use of post-quantum cryptography, it’s likely to become defacto for high security use cases in government and defence much sooner.
Organisations responsible for critical infrastructure are also likely to move more quickly – in the telco space, the GSMA, in collaboration with IBM and Vodafone, have recently launched the GSMA Post-Quantum Telco Network Taskforce. And Cloudflare has also stepped up, launching post-quantum cryptography support for all websites and APIs served through its Content Delivery Network (19+% of all websites worldwide according to W3Techs).
Importance of randomness
Irrespective of which encryption approach is adopted, their efficacy is ultimately dependent on the strength of the cryptographic key used to encrypt the data. Any weaknesses in the random number generators used to generate the keys can have catastrophic results, as was evidenced by the ROCA vulnerability in an RSA key generation library provided by Infineon back in 2017 that resulted in 750,000 Estonian national ID cards being compromised.
Encryption systems often rely upon Pseudo Random Number Generators (PRNG) that generate random numbers using mathematical algorithms, but such an approach is deterministic and reapplication of the seed generates the same random number.
True Random Number Generators (TRNGs) utilise a physical process such as thermal electrical noise that in theory is stochastic, but in reality is somewhat deterministic as it relies on post-processing algorithms to provide randomness and can be influenced by biases within the physical device. Furthermore, by being based on chaotic and complex physical systems, TRNGs are hard to model and therefore it can be hard to know if they have been manipulated by an attacker to retain the “quality of the randomness” but from a deterministic source.Ultimately, the deterministic nature of PRNGs and TRNGs opens them up to quantum attack.
A further problem with TRNGs for secure comms is that they are limited to either delivering high entropy (randomness) or high throughput (key generation frequency) but struggle to do both. In practise, as key requests ramp to serve ever-higher communication data rates, even the best TRNGs will reach a blocking rate at which the randomness is exhausted and keys can no longer be served. This either leads to downtime within the comms system, or the TRNG defaults to generating keys of 0 rendering the system highly insecure; either eventuality results in the system becoming highly susceptible to denial of service attacks.
Quantum Random Number Generators (QRNGs) are a new breed of RNGs that leverage quantum effects to generate random numbers. Not only does this achieve full entropy (i.e., truly random bit sequences) but importantly can also deliver this level of entropy at a high throughput (random bits per second) hence ideal for high bandwidth secure comms.
Having said that, not all QRNGs are created equal – in some designs, the level of randomness can be dependent on the physical construction of the device and/or the classical circuitry used for processing the output, either of which can result in the QRNG becoming deterministic and vulnerable to quantum attack in a similar fashion to the PRNG and TRNG. And just as with TRNGs, some QRNGs can run out of entropy at high data rates leading to system failure or generation of weak keys.
Careful design and robustness in implementation is therefore vital – Crypta Labs have been pioneering in quantum tech since 2014 and through their research have designed a QRNG that can deliver hundreds of megabits per second of full entropy whilst avoiding these implementation pitfalls.
Summary
Whilst time estimates vary, it’s considered inevitable that quantum computers will eventually reach sufficient maturity to beat today’s public-key algorithms – prosaically dubbed Y2Q. The Cloud Security Alliance (CSA) have started a countdown to April 14 2030 as the date by which they believe Y2Q will happen.
QKD was the industry’s initial reaction to counter this threat, but whilst meeting the security need at a theoretical level, has arguably failed to address implementation concerns in a way which is cost effective, scalable and secure for the mass market, at least to the satisfaction of NCSC and NSA.
Proponents of QKD believe key agreement and authentication mechanisms within public-key schemes can never be fully quantum-safe, and to a degree they have a point given the recent undermining of Rainbow, one of the short-listed PQC candidates. But QKD itself is only a partial solution.
The collaborative project led by NIST is therefore the most likely winner in this race, and especially given its backing by both the NSA and NCSC. Post-quantum cryptography (PQC) appears to be inherently cheaper, easier to implement, and deployable on edge devices, and can be further strengthened through the use of advanced QRNGs. Deviating away from the current public-key approach seems unnecessary compared to swapping out the current algorithms for the new PQC alternatives.
Looking to the future
Setting aside the quantum threat to today’s encryption algorithms, an area ripe for innovation is in true quantum communications, or quantum teleportation, in which information is encoded and transferred via the quantum states of matter or light.
It’s still early days, but physicists at QuTech in the Netherlands have already demonstrated teleportation between three remote, optically connected nodes in a quantum network using solid-state spin qubits.
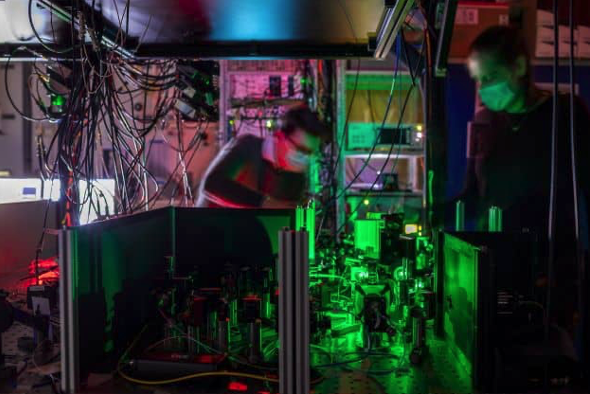
Longer term, the goal is to create a ‘quantum internet’ – a network of entangled quantum computers connected with ultra-secure quantum communication guaranteed by the fundamental laws of physics.
When will this become a reality? Well, as with all things quantum, the answer is typically ‘sometime in the next decade or so’… let’s see.
The computing landscape is one of constant change and the move to cloud has arguably been the most transformative in recent years. Early concerns around security have given way to adoption – according to Cisco’s (2022), 82% of businesses now routinely use hybrid cloud. Ironically they found it’s often security concerns driving a hybrid-cloud approach by giving teams the ability to selectively place workloads in public clouds while keeping others on-prem, or using different regions to meet data residency requirements.
But with players such as AWS, GCP and Azure creating a stranglehold on the market, there is growing awareness and a movement away from becoming too dependent on any single Cloud Service Provider (CSP), instead taking a multi-cloud approach.
Decentralisation is currently du jour in many aspects of the online world, most notably in finance (DeFi), and is starting to gain attention in the compute space through companies such as StorJ, Akash and Threefold – in essence, a blockchain-enabled approach that harnesses distributed compute & storage and will no doubt contribute to the Web3 scaffolding that underpins the future metaverse.
But decentralisation is a radical approach, and only suited to particular applications. For most enterprises today, the focus is on successfully migrating their apps into the cloud, and employing services from multiple CSPs to mitigate the dangers of becoming overly reliant on any single provider. But as many are discovering, taking a multi-cloud approach brings its own complications.
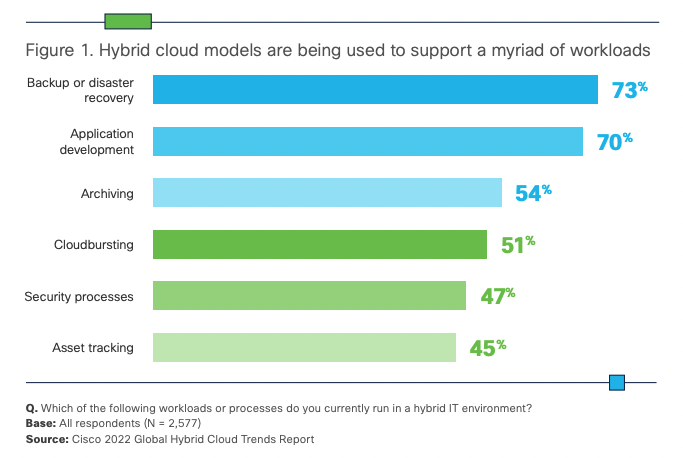
This article looks at some of the considerations and challenges that enterprises face when migrating to multi-cloud, and the resources that are out there to help them.
Cloud exuberance is over
Much has been said regarding the benefits of migrating enterprise apps to the cloud: more agility and flexibility in gaining access to resources as and when needed; an ability to scale rapidly in accordance with business needs; enabling apps hosted on-prem to burst into the cloud to accelerate workload completion time and/or generate insights with more depth and accuracy.
But it’s not all plain sailing, the hype surrounding the cloud often hides a number of drawbacks that have resulted in many businesses failing to realise the benefits expected – a recent study by Accenture Research found that only one in three companies reported achieving their cloud aims.
“Lift and shift” of legacy apps to the cloud doesn’t always work due to issues around data gravity, sovereignty, compliance, cost, and interdependencies; or perhaps because the app itself has been optimised to a specific hardware and OS used on-prem that isn’t readily available at scale in the cloud. This problem is further exacerbated by enterprises needing to move to a multi-cloud architecture.
Many believe that utilising cloud resources has a lower total cost of ownership than operating on-prem. But this doesn’t always materialise and depends on the type of systems, apps and workloads that are being considered for migration.
In the case of high performance compute (HPC) which is increasing in importance for deep learning models, simulations and complex business decisioning, enterprises running these tasks on their own infrastructure commonly dimension for high utilisation (70-90%) whereas pricing in the cloud is often orientated towards SaaS-based apps where hardware utilisation is typically <20%.
For many enterprises therefore, embarking on a programme of modernisation often results in getting caught in the middle, struggling to reach their transformation goals amidst a complex dual operating environment with some systems migrated to the cloud whilst others by necessity stay on-prem.
Optimising workloads for the cloud
For those workloads that are migrated to the cloud, delivering on the cost & performance targets set by the enterprise will be dependent on real-time analysis of workload snapshots, careful selection of the most appropriate instance types, and optimisation of the workloads to the instances that are ultimately used.
Achieving this requires a comprehensive understanding of all the compute resources available across the CSPs (assuming a multi-cloud approach), being able to select the best resource type(s) and number of instances for a given workload and SLA requirements (resilience, time, budget). In addition, where spot/pre-emptible instances are leveraged, workload data needs to be replicated between the CSPs and locations hosting the spot instances to ensure availability.
Once the target instance types are known, workload performance can be tuned using tools such as Granulate that optimise OS-level scheduling and resource management to improve performance (up to 40-60%), especially for those instances leveraging new silicon.
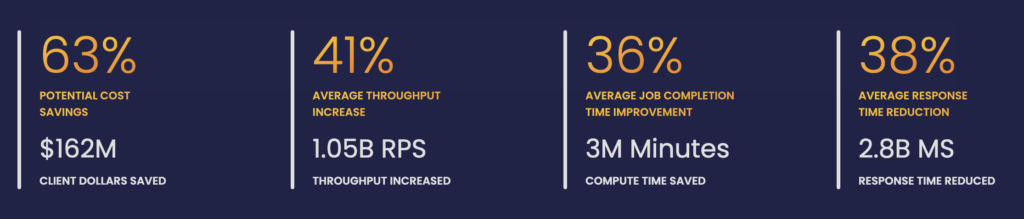
Similarly, companies such as CloudFix help enterprises ensure their AWS instances are auto-updated with the latest patches to deliver a more compliant cloud that performs better and costs less by removing the effort of applying manual fixes.
Spot instances offered by the CSPs at a discount are ideal for loosely coupled HPC workloads, and often instrumental in helping enterprises hit their targets on performance and cost; but navigating the vast array of instance types and pricing models is far from trivial.
Moreover, prices often fluctuate based on demand, availability, and region. 451 Research’s Cloud Price Index (CPI) for instance recorded more than 1.2 million service changes in 2021 (SKUs added, SKUs removed, price increases and price decreases).
So whilst spot instances can help with budgetary targets and economic viability for HPC workloads, juggling instances to optimise cost and break-even point between reserved instances, on-demand, and spot/preemptible instances, versus retaining workloads on-prem, can become a real challenge for teams to manage.
Furthermore, with spot prices fluctuating frequently and resources being reclaimed with little notice by the CSP, teams need to closely monitor cloud usage, throttling down workloads when pricing rises above budget, migrating workloads when resources are reclaimed, and tearing down resources when they’re no longer needed. This can soon become an operational and administrative nightmare.
Cloud Management Platforms
Cloud Management Platforms (CMP) aim to address this with a set of tools for streamlining operations and enabling cloud resources to be utilised more effectively.
Whilst it’s true that CSPs provide such tools to aid their customers (such as AWS CloudWatch), they are proprietary in nature and vary in functionality, complicating the situation for any enterprise with multi-cloud deployments – in fact, Cisco found that a third of responding organisations highlighted operational complexity as a significant concern when adopting hybrid or multi-cloud models.
This is where CMPs come in, providing a “unified” experience and smoothing out the differences when working with multiple CSPs.
Such platforms provide an ability to:
- create clusters of mixed instance types to avoid provider lock-in and circumvent constraints imposed by any single CSP when dealing with large clusters
- manage workloads and resource provisioning simultaneously to maximise efficiency
- track progress and scheduling of all workloads via a single dashboard
- dynamically change prioritisation or allocation of resources to deliver results on time and within budget
- monitor when workloads start and complete to ensure that resources are not left running
CMPs achieve this by leveraging the disparate resources and tooling of the respective cloud providers to deliver a single homogenised set of resources for use by the enterprise’s apps.
Moreover, by unifying all elements of provisioning, scheduling and cost management within a single platform, they enable a more collaborative working relationship between teams within the organisation (FinOps). FinOps has demonstrated a reduction in cloud spend by 20-30% by empowering individual teams to manage their cloud usage whilst enabling better alignment with business metrics and strategic decision-making.
Introducing YellowDog
YellowDog is a leader in the CMP space with a focus on enterprises seeking a mix of public, private and on-prem resources for HPC workloads.
In short, the YellowDog platform combines intelligent orchestration, scheduling and dynamic policy-driven provisioning at scale across on-prem, hybrid and multi-cloud environments using agent technology. The platform has applicability ranging from containerised workloads through to supporting bare-metal servers without a hypervisor.
Compute resources are formulated into “on-demand” clusters and abstracted through the notion of workers (threads on instances). YellowDog’s Workload Manager is a cloud native scheduler that scales beyond existing technologies to millions of processor cores, working across all the CSPs, multi-region, multi-datacentre and multi-instance shapes.
It can utilise Spot type instances where others can’t, acting as both a native scheduler and meta-scheduler (invoking 3rd party technologies and creating specific workload environments such as Slurm, OpenMPI etc.) to work with both loosely coupled and tightly coupled workloads.
YellowDog’s workload manager matches workload demand with the supply of workers whilst ensuring compatibility (via YellowDog’s extensive Image Registry) and automatically reassigning workloads in case of instance removal – effectively it is “self-healing”, automatically provisioning and deprovisioning instances to match the workload queue(s).
The choice of which workers to choose is managed by the enterprise through a set of compute templates defining workload specific compute requirements, data compliance restrictions and enterprise policy on use of renewables etc. Compute templates can also be attribute-driven via live CSP information (price, performance, geographic region, reliability, carbon footprint etc.), and potentially in future with input from CPU and GPU vendors (e.g., to help optimise workloads to new silicon).
On completion, workload output can be captured in YellowDog’s Object Store Service for subsequent analysis and collection or as input to other workloads. By combining multiple storage providers (e.g. Azure Blob, Amazon S3, Google Cloud Storage) into one coherent data surface, YellowDog mitigate the issue of data gravity and ensure that data is in the right place for use within a workload. YellowDog also supports the use of other file storage technologies (e.g. NFS, Lustre, BeeGFS) for data seeding and management.
In addition, the enterprise can define pipelines that are automatically triggered when a new file is uploaded into an object store that spin up instances and work on the new file, and then shut down when the work is completed.
As jobs are running, YellowDog enables different teams to monitor their individual workloads with real-time feedback on progress and status, as well as providing an aggregate view and ability to centrally manage quotas or allowances for different clouds, users, groups and so forth.
In summary
Multi-cloud is becoming the norm, with businesses typically using >2 different providers. In fact Cisco found that 58% of those surveyed use 2-3 CSPs for their workloads, with 31% using more than 4. Effective management of these multi-cloud environments will be paramount to ensuring future enterprise growth. Cloud Management Platforms, such as that offered by YellowDog, will play an important role in helping enterprises to maximise their use of hybrid / multi-cloud.
The focus of AI and ML innovation to-date has understandably been in those areas characterised by an abundance of labelled data with the goal of deriving insights, making recommendations and automating processes.
But not every potential application of AI produces enough labelled data to utilise such techniques – use cases such as spotting manufacturing defects on a production line is a good example where images of defects (for training purposes) are scarce and hence a different approach is needed.
Interest is now turning within academia and AI labs to the harder class of problems in which data is limited or more variable in nature, requiring a different approach. Techniques include: leveraging datasets in a similar domain (few-shot learning), auto-generating labels (semi-supervised learning), leveraging the underlying structure of data (self-supervised learning), or even synthesising data to simulate missing data (data augmentation).
Characterising limited-data problems
Deep learning using neural networks has become increasingly adept at performing tasks such as image classification and natural language processing (NLP), and seen widespread adoption across many industries and diverse sectors.
Machine Learning is a data driven approach, with deep learning models requiring thousands of labelled images to build predictive models that are more accurate and robust. And whilst it’s generally true that more data is better, it can take much more data to deliver relatively marginal improvements in performance.

Figure 1: Diminishing returns of two example AI algorithms [Source: https://medium.com/@charlesbrun]
Manually gathering and labelling data to train ML models is expensive and time consuming. To address this, the commercial world has built large sets of labelled data, often through crowd-sourcing and through specialists like iMerit offering data labelling and annotation services.
But such data libraries and collection techniques are best suited to generalist image classification. For manufacturing, and in particular spotting defects on a production line, the 10,000+ images required per defect to achieve sufficient performance is unlikely to exist, the typical manufacturing defect rate being less than 1%. This is a good example of a ‘limited-data’ problem, and in such circumstances ML models tend to overfit (over optimise) to the sparse training data, hence struggle to generalise to new (unknown) images and end up delivering poor overall performance as a result.
So what can be done for limited-data use cases?
A number of different techniques can be used for addressing these limited-data problems depending on the circumstances, type of data and the amount of training examples available.
- Few-shot learning
Few-shot learning is a set of techniques that can be used in situations where there are only a few example images (shots) in the training data for each class of image (e.g. dogs, cats). The fewer the examples, the greater the risk of the model overfitting (leading to poor performance) or adversely introducing bias into the model’s predictions. To address this issue, few-shot learning leverages a separate but related larger dataset to (pre)train the target model.
Three of the more popular approaches are meta-learning (training a meta-learner to extract generalisable knowledge), transfer learning (utilising shared knowledge between source and target domains) and metric learning (classifying an unseen sample based on its similarity to labelled samples).
Once a human has seen one or two pictures of a new animal species, they’re pretty good at recognising that animal species in other images – this is a good example of meta-learning. When meta-learning is applied in the context of ML, the model consecutively learns how to solve lots of different tasks, and in doing so becomes better at learning how to handle new tasks; in essence, ‘learning how to learn’ similar to a human – illustrated below:
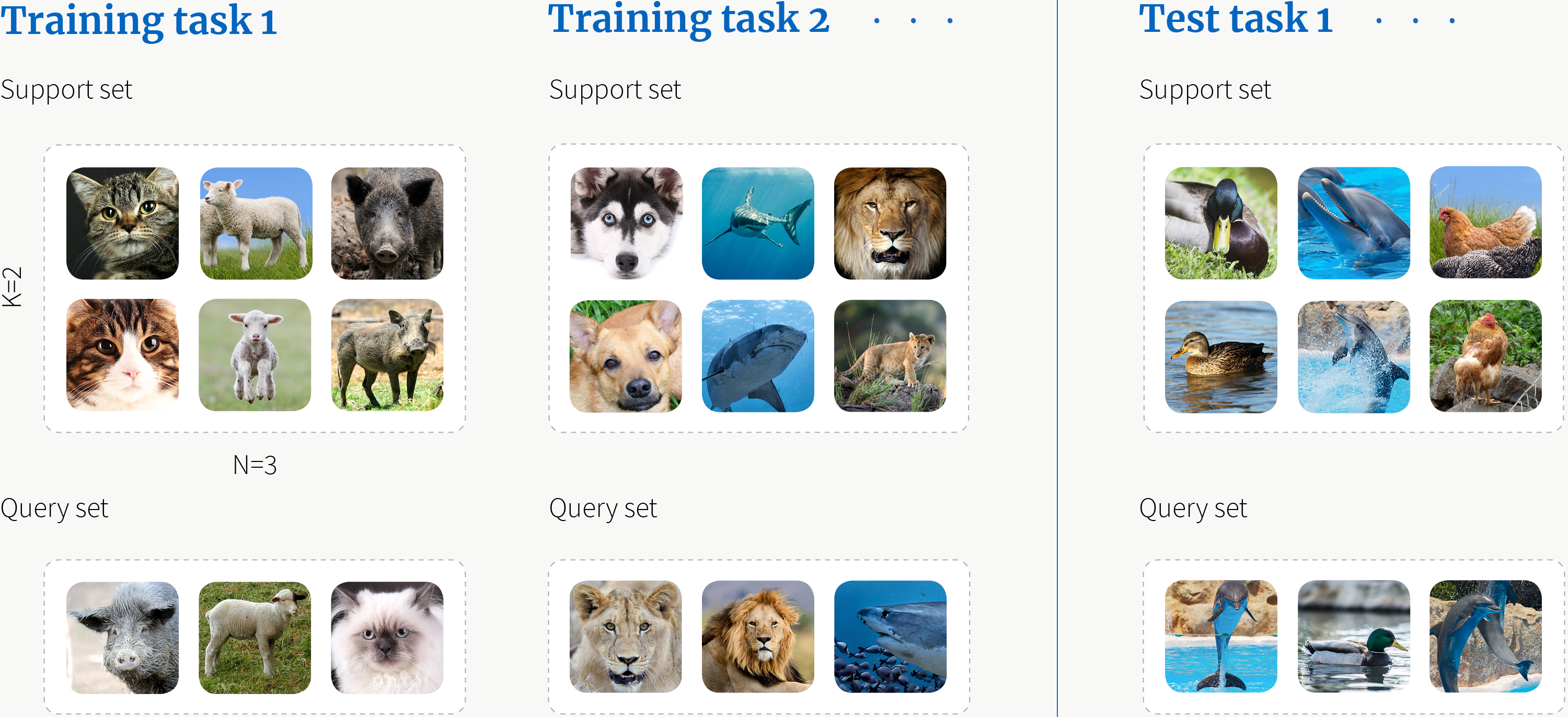
Figure 2: Meta-learning [Source: www.borealisai.com]
Transfer learning takes a different approach. When training ML models, part of the training effort involves learning how to extract features from the data; this feature extraction part of the neural network will be very similar for problems in similar domains, such as recognising different animal species, and hence can be used in instances where there is limited data.
Metric learning (or distance metric learning) determines similarity between images based on a distance metric and decides whether two images are sufficiently similar to be considered the same. Deep metric learning takes the approach one step further by using neural networks to automatically learn discriminative features from the images and compute the distance metric based on these features – very similar in fact to how a human learns to differentiate animal species.
- Self-supervised & semi-supervised learning
Techniques such as few-shot learning can work well in situations where there is a larger labelled dataset (or pre-trained model) in a similar domain, but this won’t always be the case.
Semi-supervised learning can address this lack of sufficient data by leveraging the data that is labelled to predict labels for the rest hence creating a larger labelled dataset for use in training. But what if there isn’t any labelled data? In such circumstances, self-supervised learning is an emerging technique that sidesteps the lack of labelled data by obtaining supervisory signals from the data itself, such as the underlying structure in the data.
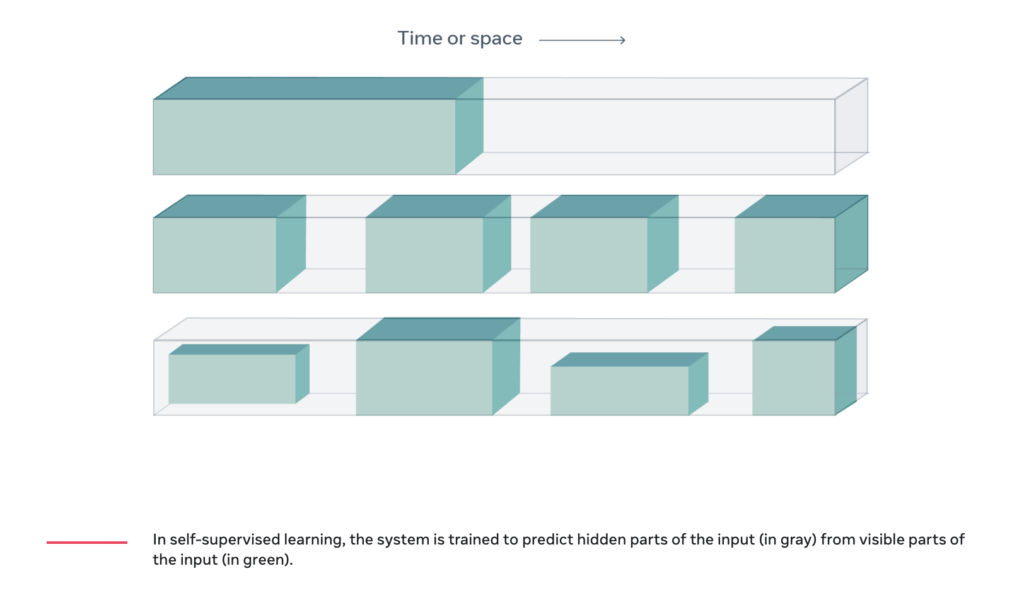
Figure 3 Predicting hidden parts of the input (in grey) from visible parts (in green) using self-supervised learning [source: metaAI]
- Data augmentation
An alternate approach is simply to fill the gap through data augmentation by simulating real-world events and synthesising data samples to create a sufficiently large dataset for training. Such an approach has been used by Tesla to complement the billions of real-world images captured via its fleet of autonomous vehicles for training their AI algorithms, and by Amazon within their Amazon’s Go stores for determining which products each customer is taking from the shelves.
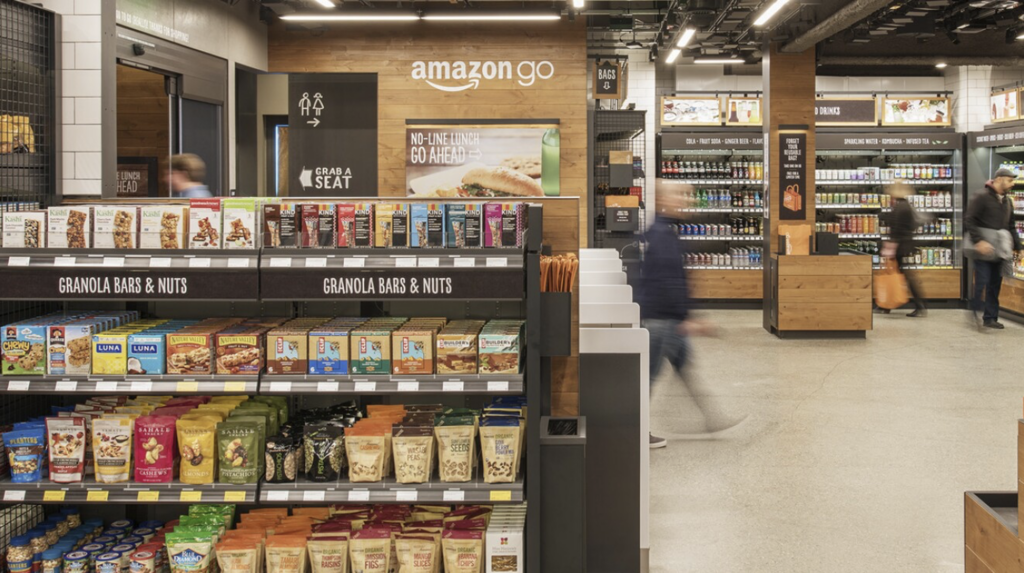
Figure 4: An Amazon Go store [Source: https://www.aboutamazon.com/what-we-do]
Whilst synthetic data might seem like a panacea for any limited-data problem, it’s too costly to simulate for every eventuality, and it’s impractical to predict anomalies or defects a system may face when put into operation.
Data augmentation has the potential to reinforce any biases that may be present in the limited amount of original labelled data, and/or causing overfitting of the model by creating too much similarity within the training samples such that the model struggles to generalise to the real-world.
Applying these techniques to computer vision
Mindtrace is utilising the unsupervised and few-shot learning techniques described previously to deliver a computer vision system that is especially adept in environments characterised by limited input data and where models need to adapt to changing real-life conditions.
Pre-trained models bringing knowledge from different domains create a base AI solution that is fine-tuned from limited (few-shot) or unlabelled data to deliver state-of-the-art performance for asset inspection and defect detection.
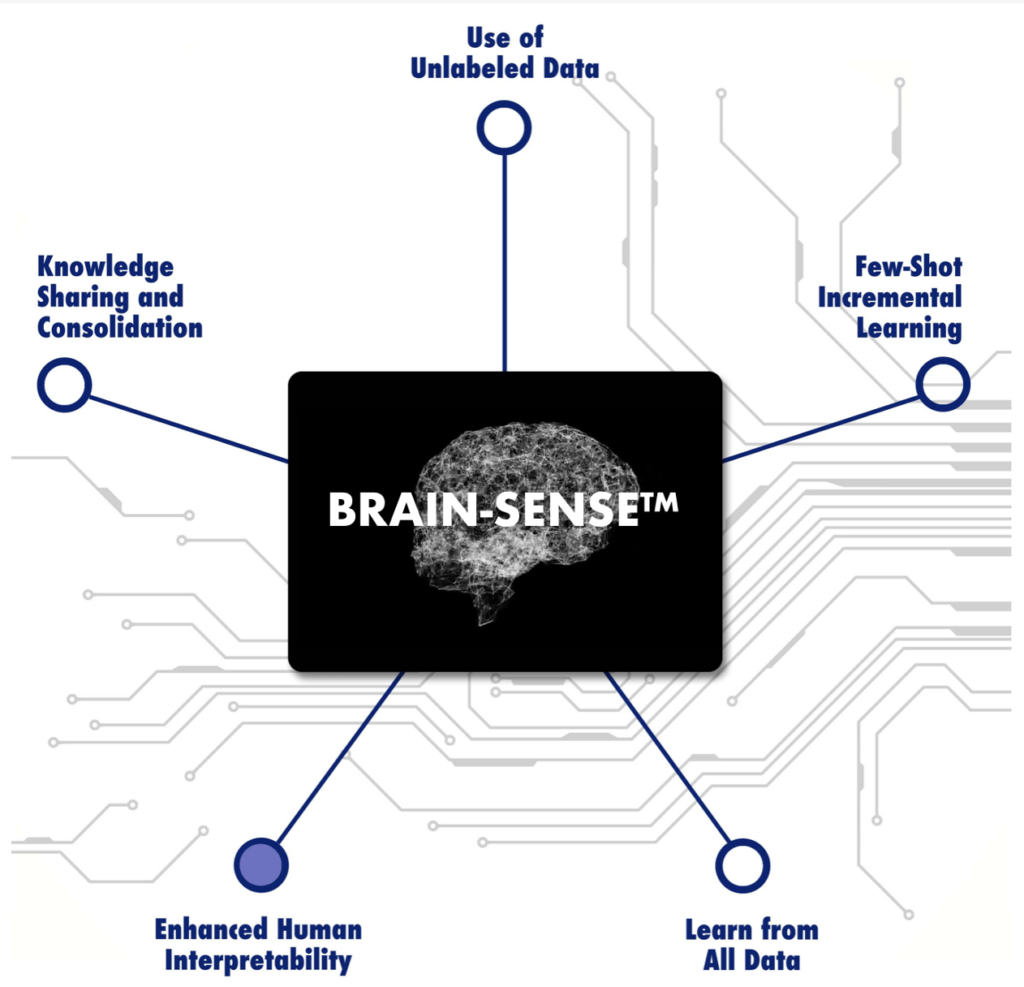
Figure 6: Mindtrace [Source: https://www.mindtrace.ai]
This approach enables efficient learning from limited data, drastically reducing the need for labelled data (by up to 90%) and the time / cost of model development (by a factor of 6x) whilst delivering high accuracy.
Furthermore, the approach is auto-adaptive, the models continuously learn and adapt after deployment without needing to be retrained, and are better able to react to changing circumstances in asset inspection or new cameras on a production line for detecting defects, for example.
The solution is also specifically designed for deployment at the edge by reducing the size of the model through pruning (optimal feature selection) and reducing the processing and memory overhead via quantisation (reducing the precision using lower bitwidths).
Furthermore, through a process of swarm learning, insights and learnings can be shared between edge devices without having to share the data itself or process the data centrally, hence enabling all devices to feed off one-another to improve performance and quickly learn to perform new tasks (Bloc invested in Mindtrace in 2021).
In summary
The focus of AI and ML innovation to-date has understandably been in areas characterised by an abundance of labelled data to derive insights, make recommendations or automate processes.
Increasingly though, interest is turning to the harder class of problems with data that is limited and dynamic in nature such as the asset inspection examples discussed. Within Industry 4.0, limited-data ML techniques can be used by autonomous robots to learn a new movement or manipulation action in a similar way to a human with minimal training, or to auto-navigate around a new or changing environment without needing to be re-programmed.
Limited-data ML is now being trialled across cyber threat intelligence, visual security (people and things), scene processing within military applications, medical imaging (e.g., to detect rare pathologies) and smart retail applications.
Mindtrace has developed a framework that can deliver across a multitude of corporate needs.
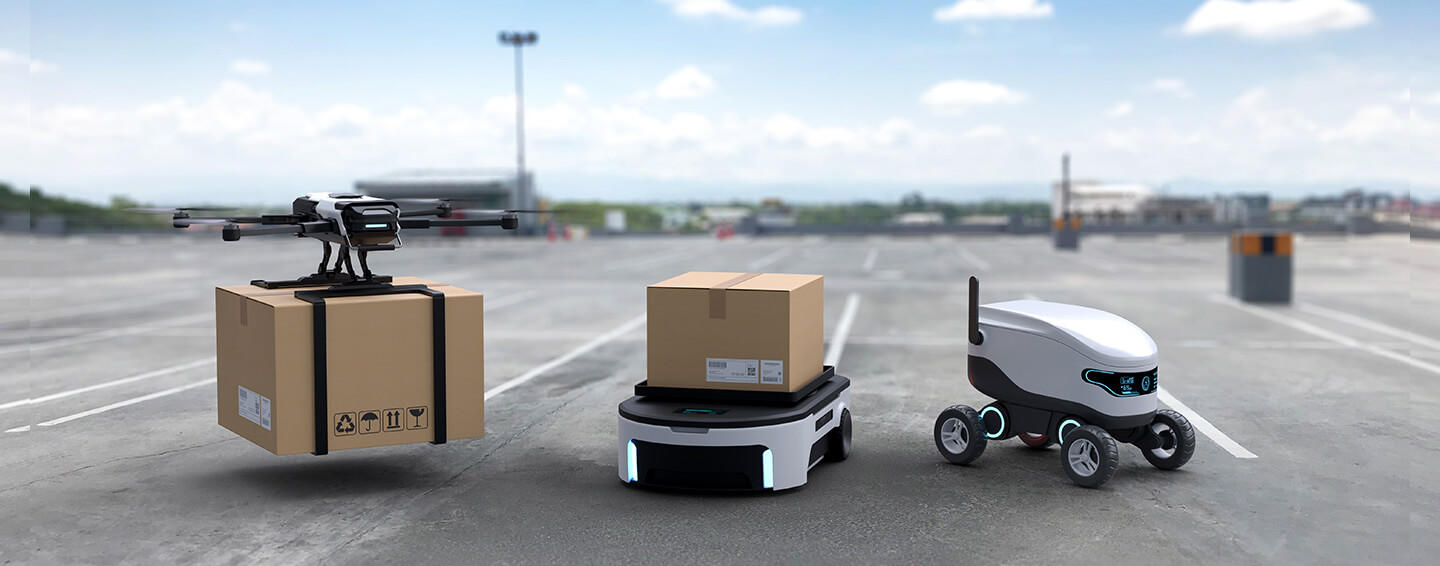
Figure 7: Example Autonomous Mobile Robots from Panasonic [Source: Panasonic]
Industry 4.0 driving the need for 5G
Automation in Industry 4.0 sectors such as smart manufacturing, warehousing, mining and ports is driving increased demand for high performance connectivity. Wi-Fi is widely deployed today but is limited in terms of reliability and support for critical mobility use cases – 5G is much better placed to meet these needs.
In particular, 5G can meet requirements around high bandwidth and low latency, whilst also delivering resiliency through dedicated radio spectrum and has the flexibility to support full mobility ranging from indoor use to wide area outdoor coverage.
A common misconception is that many of these benefits are available within Wi-Fi 6, but whilst Wi-Fi 6 can offer high capacity, it can’t manage radio resources as efficiently as 5G and is intrinsically hampered by sharing unlicensed spectrum, whilst 5G using dedicated spectrum is inherently more reliable.
It would also be missing the point to say that 5G is simply a ‘faster 4G’ – 5G adopts a service-based architecture (SBA) which enables provisioning of customised network slices and zero-touch network operations that provides much finer granularity in how a 5G network can be set up and run.
5G is therefore growing in favour, 75% of manufacturers indicating that 5G is a key enabler within their digital transformation strategies [Capgemini’s global enterprise 5G survey].
Nevertheless, it’s not a clear homerun for 5G and to succeed it must provide the best of both worlds – the functionality, performance and reliability of 5G, twinned with the flexibility, control and ease of use of Wi-Fi deployments.
Delivering 5G to meet enterprise needs
- Option 1: a public 5G network slice
Network slicing is a new capability introduced in 5G that enables mobile network operators (MNOs) to leverage their public 5G infrastructure to provide virtualised private networks to enterprises.
A number of slice types have been defined within the 3GPP standards (3GPP TS23.501):
- eMBB (enhanced mobile broadband) – for applications requiring stable connections with very high peak data rates
- URLLC (ultra-reliable low latency communications) – for applications that have strict reliability and latency requirements such as industrial automation and autonomous vehicles (i.e., devices requiring mission critical connectivity)
- mMTC (massive machine-type communications) – to support a massive number of IoT devices within a defined area which are only sporadically active in sending small data payloads (e.g., sensors)
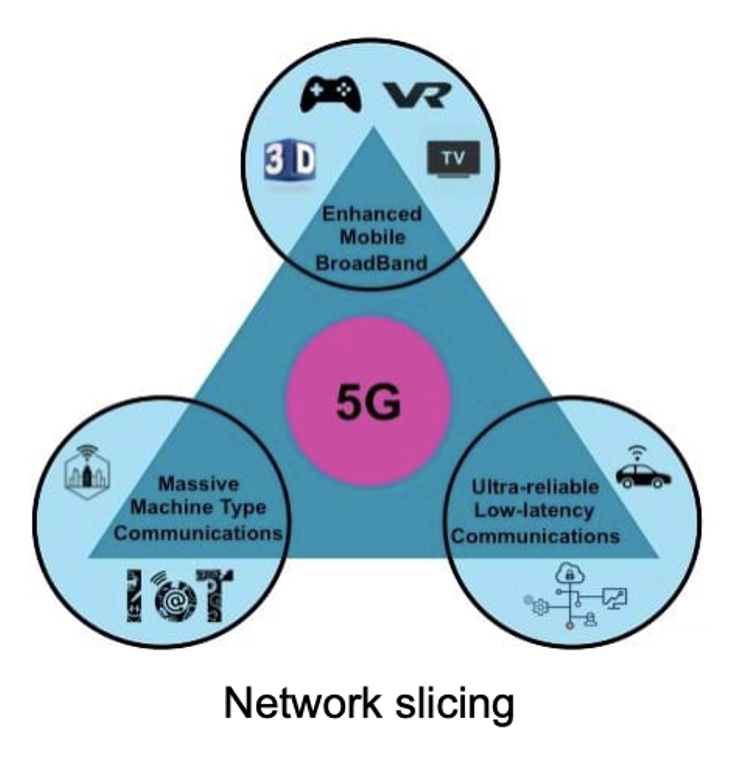
In a manufacturing example, a computer vision system used for monitoring a production line may require consistent throughput with an ultra-reliable connection and be best served by a URLLC slice, whilst sensors for monitoring humidity levels may only need to connect intermittently to send signals to a control centre and be adequately served using an mMTC slice.
But this approach may be too constraining for some enterprises – the slices being statically defined, whereas what many enterprises really want is the ability to control their connectivity on a more dynamic basis to map resources to an application as circumstances change (adaptive slicing).
As 5G public networks evolve towards fully cloud-native architectures, it will become possible to provision highly customised network slices tailored to specific services. But for now, MNO public 5G offerings are limited by the current approach of predefined eMBB, URLLC, and mMTC slices.
Given these constraints, enterprises are increasingly exploring the option of procuring their own 5G mobile private network (MPN) that can be tailored specifically to their needs.
- Option 2: a 5G mobile private network (MPN)
A 5G MPN is a 5G network (RAN and 5G core) that has been designed, configured and deployed specifically for a given enterprise customer.
Mobile networks are designed to utilise specific licensed spectrum, so the logical choice would be to procure an MPN from an MNO. But with the introduction of shared spectrum in many countries (including the UK) and open flexible architectures (via OpenRAN) there are now many new entrants entering the space offering solutions to enterprises either direct or through partnership.
This gives enterprises the flexibility to decide whether to go with a Managed Service Provider (MSP) that can fully design, deploy, configure and optionally operate the MPN for them (e.g., a school campus), or work with a selection of vendors and partners to assemble their own MPN infrastructure tailored to their requirements (e.g., smart manufacturing, ports, mining etc.).
Currently, all options and potential partnerships are being explored in the marketplace.
MNOs and incumbents such as Ericsson and Nokia are partnering to bring MPN propositions to their enterprise client base (e.g., Ericsson Industry Connect). But equally MNOs are also partnering up with challengers (Affirmed Networks, Parallel Wireless, Metaswitch, Mavenir, Celona et al) and leveraging cloud resources (e.g., Azure, AWS Wavelength) and enterprise IT partners (Cisco, IBM, Oracle) to increase their flexibility and agility in bringing solutions to market that encompass not only connectivity but also provide the cloud, edge and AI capabilities needed by enterprises for their end-end application delivery.
Whilst the necessity of acquiring licensed spectrum for 5G MPN deployments drives many of these players into partnering with the MNOs, in those markets where shared spectrum has been allocated, these players are also able to step up, adopt the role of a Managed Service Provider, and offer complete MPN solutions directly to enterprise clients. Nokia, Ericsson, Mavenir, Celona, Federated Wireless, Expeto and many more all have direct-to-market propositions, and the hyperscalers are also eying up the opportunity with both Amazon and more recently Google announcing MPN offerings, either developed in-house or through partnership (Google working with Betacom, Boingo, Celona and Kajeet in the US).
Enterprises are faced with many options, but this also gives them huge flexibility in finding the best match for their functional and operational needs and also affords them with higher levels of privacy by operating the infrastructure themselves rather than sharing infrastructure within a public network – for those in manufacturing, high security is a key driver in choosing an MPN over utilising a public 5G network slice.
Given the opportunity, it’s hardly surprising that deploying private 5G is a top priority now for IT decision makers in enterprises [Technalysis Research] and 76% of those in manufacturing plan to deploy 5G MPNs by 2024 [Accedian].
Optimising connectivity to match use cases
A key attraction for enterprises in deploying their own 5G MPN is the flexibility it gives them in optimising connectivity to match application requirements. This can be achieved through the definition of an ‘intent’ that states expectations on service delivery and network operation through the expression of a set of goals, functional requirements, and constraints.
The table below describes the requirements for example use cases within a factory automation context:
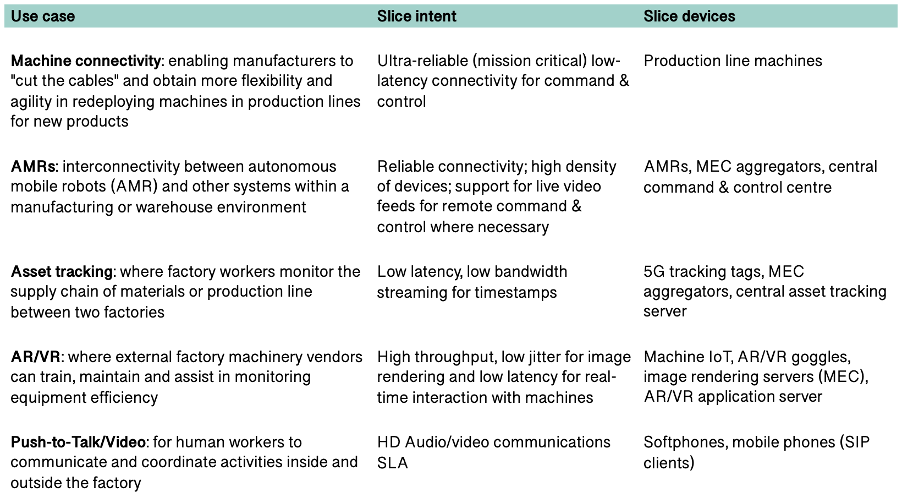
At a practical level, intents can be managed in a number of ways depending on the skillsets of the enterprise. For those enterprises with limited expertise, a set of low/no-code tools can be provided for defining intents, app/device group administration, and monitoring network and application performance as well as end-end security.
Conversely, for those wanting more fine-grained control, orchestration could be provided to DevOps teams through RESTful APIs with dynamic control over throughput, latency, packet error rate metrics, network segments / IP domains etc., and/or bootstrapped via Infrastructure as Code (IaC) templates – in short, the aim is to enable enterprises to configure and manage their 5G MPNs using DevOps-friendly interfaces as easily as Kubernetes enables them to do with cloud resources for their application and services.
Introducing Zeetta
Zeetta delivers on this vision by hiding the details of vendors and technology domains under a layer of abstraction and then enabling the enterprise application developers to consume these services in an end-to-end low/no-code fashion. This application-centric, end-to-end view also enables DevOps teams to independently innovate and operate applications without the need for centralized large networking groups.
The platform has been developed and trialled within the £9m 5G-ENCODE project, and provides enterprises with a ‘single pane of glass’ to visualise their end-to-end network as well as a set of automation features for optimal network management:
Automate
- Automates the design, scheduling and provisioning of network slices in line with intents specifying connectivity and QoS requirements; intents can be predefined or can be modelled; public 5G slices can also be sub-sliced through the use of a policy-based scheduler to provide more fine-grained control in multiplexing multiple applications over a single slice resource
Adapt
- Facilitates performance monitoring of each network slice, flagging any deviations from the targeted intent and helping the enterprise team to determine the root cause and remediate, e.g., by adapting a slice as needed to best serve the affected application
- Similarly, automates fault isolation hence speeding issue resolution and delivering a better overall MPN quality & robustness
Accelerate
- Modifies application intent where needed to keep pace with varying application requirements as circumstances change
Zeetta translates the demand and intent into a set of parameters and complex actions for each domain, and leverages the open interfaces provided by the MNOs/MSPs supplying the MPN to create the connectivity slice and avoid over-dimensioning of the RAN, Core and BSS/OSS hence reducing cost (CAPEX and OPEX). This slice is then continuously monitored, compared and adapted based on the quality of experience (QoE) targets.
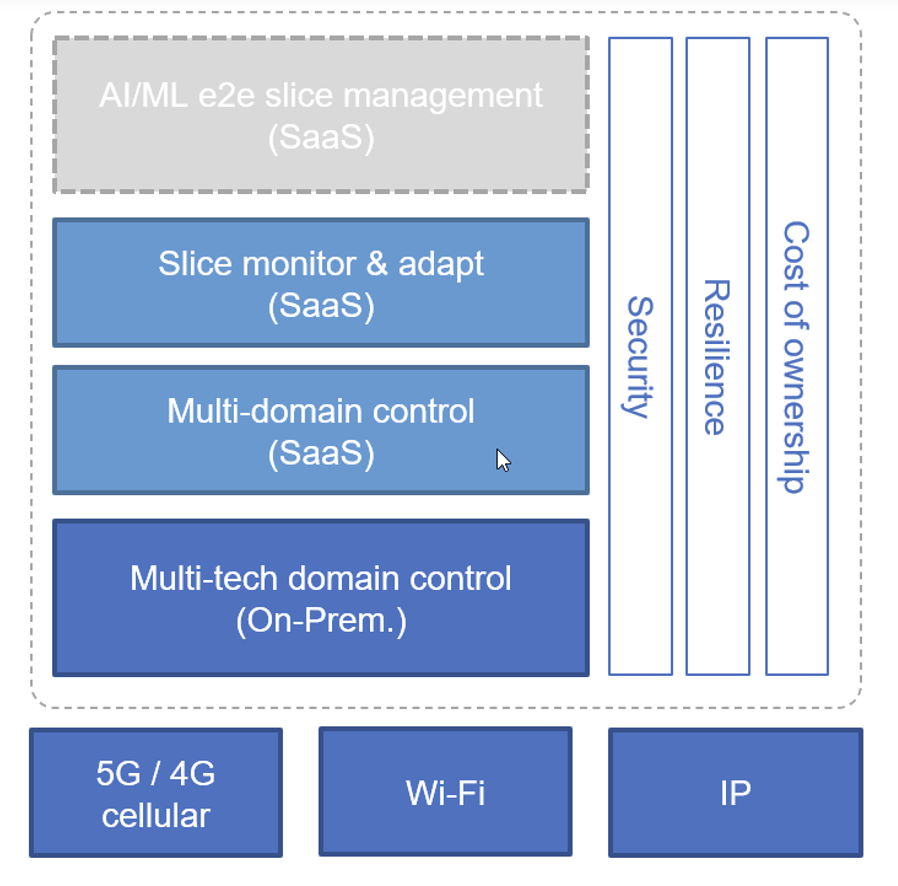
Zeetta product architecture
In summary
5G offers high capacity, low latency, and full flexibility, coupled with reliability through dedicated spectrum. Whilst public 5G network slices will evolve over time, the current lack of in-building coverage and fine-grained control means that for many enterprises the best solution is to procure their own 5G MPN.
Many pilots [Vodafone & Ford] have already demonstrated the significant benefits of 5G MPNs and a number of initial deployments are already operational [Verizon & UK ports]. 5G MPN rollout is likely to reach around 25k installations by 2026 and accelerate rapidly to ~120k by 2030 [Analysis Mason; IDC; Polaris Market Research; ABI research].
Whilst many have leant heavily on MNOs to help design, deploy and configure their MPNs, such an approach will be difficult to scale, and the growth projections are unlikely to be realised unless 5G MPNs can be as simple to deploy and manage as experienced with cloud resources today.
If achieved, this will open up 5G MPNs to enterprises of all sizes – in essence, similar to the democratisation of telco APIs brought about by the introduction of developer-friendly platforms (and RESTful APIs) from the likes of Twilio a decade or so ago.
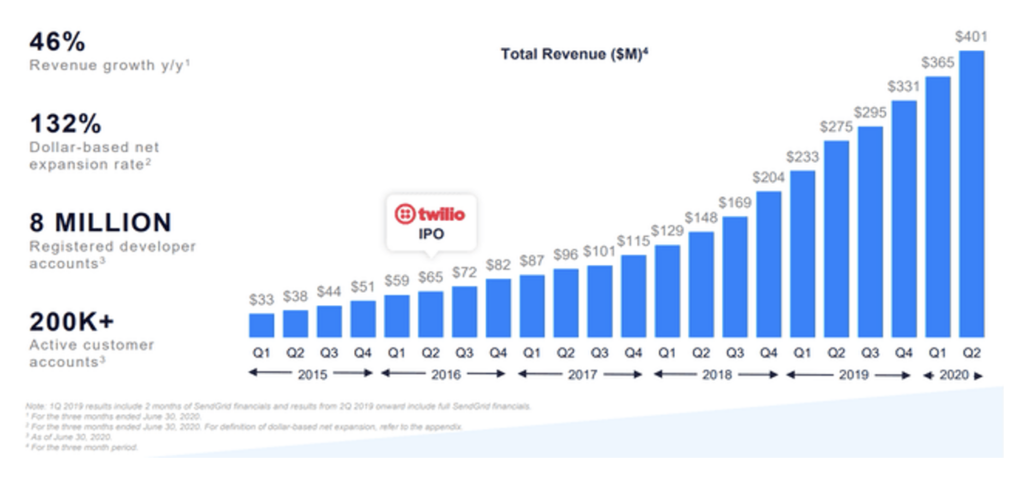
Twilio growth in the past decade [source: Twilio]
The cloud emerged in a similar timeframe, but since those early launches of elastic processing and storage, a multibillion-dollar industry has grown up around them supplying tools and supplementary services to make the consumption of these resources simpler. To enable enterprise 5G MPNs to be built on-demand as simply as is now enjoyed with cloud resources will require a similar ecosystem of tools and services to emerge.
Zeetta is leading the vanguard in this regard by providing a sophisticated orchestration tool that acts essentially as a ‘Kubernetes for MPNs’, but extends across multiple technology domains (4G, 5G, Wi-Fi, SD-WAN, MEC, public 5G slices etc.) to provide comprehensive management, and all exposed via an intuitive ‘single pane of glass’ and DevOps-friendly interface.
Demand for high performance compute (HPC) on the rise
Once the stalwart of particle physicists, Formula 1 designers, and climate forecasters, the demand for HPC is rapidly going mainstream as corporates increasingly introduce deep learning models, simulations and complex business decisioning into their daily operations.
HPC can play a pivotal role in accelerating product design, tackling complex problems and enabling businesses to generate insights faster and with more depth and accuracy, and has applicability across an ever-widening range of industries including financial services, media, gaming and retail.
To-date, the only option for corporates seeking to access this level of compute was to build, maintain and operate dedicated HPC facilities in-house, but this brings a number of challenges. The first is cost – HPC systems are expensive, and only around 7% of the budget actually goes toward the hardware, the rest being consumed by buildings, staffing, power, cooling, networking etc. Moreover, because many of these systems are designed to support peak demand, utilisation can be as little as 60% for the majority of the time.
What’s more, significant additional capital is needed on a three year upgrade cycle to keep pace with demand as the business grows and the volume and complexity of workloads increases, and/or to reap the benefits of the latest computing technology. But this computing resource is intrinsically finite and hence projects within an organisation need to be prioritised leading to many missing out or having to step aside if more urgent tasks come along.
HPC on-premise deployments are traditionally designed and optimised around particular use cases (such as climate forecasting) whereas corporates today need HPC resources that can support a much broader set of applications and be able to adapt as workload characteristics evolve in reaction to the fast-moving competitive landscape.
Many companies are therefore turning to cloud-based HPC.
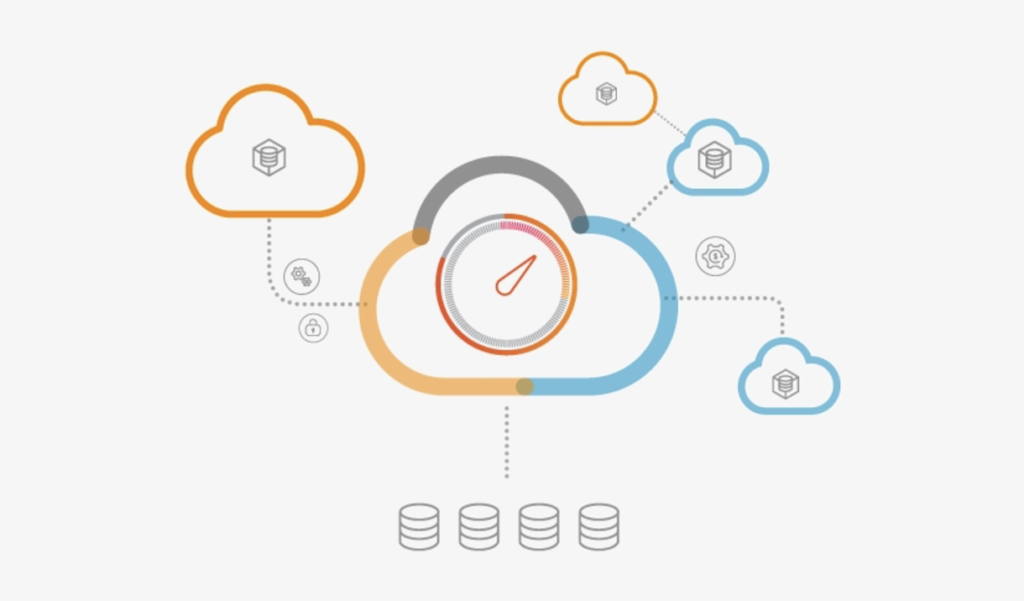
[Image Source: www.seekpng.com]
Benefits of cloud HPC
First and foremost, cloud HPC provides more flexibility for an organisation to gain access to HPC resources as and when needed and scale to match individual workload demands.
It also opens up more choices for the corporate; for instance, employing 10x HPC resources to accelerate product design and gain competitive advantage by being first to market. Or increasing productivity by removing compute barriers so that the corporate can use more detailed simulations or eliminate the effort in simplifying deep learning models to fit inside legacy hardware.
A cloud-based approach mitigates the risk in cost & complexity of operating HPC on-prem by providing flexibility to manage the cost/performance trade-off, allowing HPC environments to be created on the fly and then torn down as soon as the workloads have completed to avoid the corporate paying for resources and software licenses that are no longer needed.
To accommodate this variability in customer demand, CSPs dimension their cloud infrastructure with excess capacity which is powered-up and ready to use but otherwise sitting idle. To offset the monetary and environmental impact of this idle infrastructure, CSPs offer this excess capacity in the form of preemptible instances at massive discounts (up to 90% in some cases) but with the caveat that the resource can be reclaimed by the CSP at a moment’s notice if required by a full-paying customer – a corporate choosing to use these preemptible instances is essentially trading availability guarantees for a variable but much reduced ‘Spot’ price.
HPC workloads such as running a simulation, training a deep learning model, analysing a big data set or encoding video are periodic and batch in nature and not dependent on continuous availability hence a good fit for preemptible resources. If some of the resource instances within the HPC cluster are reclaimed during processing, the workload slows but does not completely stop. Ideally though, it should be possible to quickly and seamlessly re-distribute the part of the workload that was interrupted to alternate resources at the same or a different CSP thereby ensuring that the workload still completes on time – this is possible but not easy, and an area of speciality for some 3rd party tool providers.
With the increase in availability of HPC resources twinned with the ability to closely manage cost, corporates get the opportunity to open up HPC resources to the wider organisation, enabling a wider range of teams, departments and geographically dispersed business units to access the processing power they need whilst being able to track cost and performance and focus on outcomes rather than managing operational complexity.
In a world that is speeding up, becoming more competitive, and being driven by continuous integration and continuous delivery (CI/CD), easy access to cost-effective HPC resources on-the-fly is likely to become a key requirement for any corporate wishing to stay ahead.
Considerations when leveraging cloud HPC
Running complex technical workloads in the cloud is not as simple as swiping a credit card and getting a cloud account.
Many of the companies coming to cloud HPC will be specialists in their area, and may also have cloud expertise, but will need support in composing their workloads to take advantage of the parallelism within the cloud HPC stack, and tools to help them optimise their use of cloud resources.
Such tools will need to work across both workload management and resource provisioning, balancing them to meet the corporate’s target SLAs whether that be dynamically adding more resource to complete a workload on time, or prioritising and scheduling workloads to make most effective use of resources to meet budgetary constraints.
More specifically, tools will be needed that can:
Conduct realtime analysis of workload snapshots to determine their compute requirements.
Sift through the bewildering array of 30,000 different compute resources offered by the CSPs to ensure the best fit for each individual workload whilst also abiding by any corporate policy or individual budgetary targets. Factors that may need to be taken into account when selecting appropriate resources include:
- Workloads that are limited by the number of cores they can use
- Workloads that require particular processor hardware to match the OS used within a virtual image
- Workloads that may have scaling limitations due to the nature of the application licensing model provided by the software provider(s) that may not allow for bursting above a small number of processors
- Workloads that require processor hyper-threading to be disabled and/or are dependent on bare metal servers as opposed to virtual machines to maximise performance
- Tightly-coupled workloads that have specific latency and bandwidth requirements for communication between the cluster nodes
- Workloads involving sensitive data or regulatory restrictions that require all processing to be conducted within a particular locale
- Preference for cloud resources powered via renewables to meet corporate ESG targets
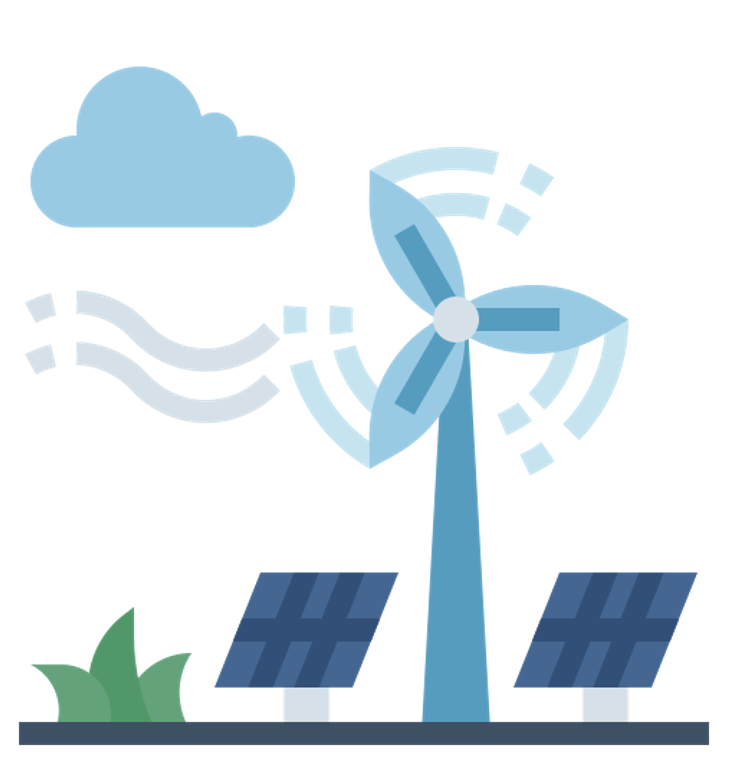
Create clusters of mixed instance types, and do this x-CSP to avoid vendor lock-in and/or to circumvent constraints imposed by any single CSP when dealing with large clusters.
Ensure the workload data is available in the relevant cloud by replicating data between CSPs and locations to ensure availability should a workload need to be executed there.
Monitor when workloads start and complete to ensure that resources are not left running when no workloads are executing.
Intelligently monitor spot/preemptible instances (where used) to ensure that workload cost stays within budget as the spot pricing fluctuates with demand, and reallocate workloads seamlessly if instances are reclaimed by the CSP to ensure that the composite cluster is able to deliver against the workload targets.
Integrate into a corporate’s DevOps and CI/CD processes to enable accessibility of HPC resources more broadly across the organisation.
Provide a single view of workload status and enable users to dynamically make changes to their workloads to deliver results on time and within their project budgetary constraints.
Coordinate with any 3rd party schedulers already used by the corporate (e.g., Slurm, IBM LSF, TIBCO DataSynapse GridServer etc.) to provide a single meta system for workload submission and management across on-prem, fixed cloud and public cloud HPC resources.
Client types and associated requirements
The relative importance of these different tools and requirements will very much be determined by the type of company seeking to utilise cloud HPC, the level of resources they may already have in place and the type of workloads they need to support.
Three example client types are outlined:
HPC stalwarts
Multi-national organisations and specialist corporates in sectors such as academia, engineering, life sciences, oil & gas, aircraft and automotive that already have an HPC data centre on-prem but aim to supplement it with cloud HPC resources to avoid the cost of building out and maintaining additional HPC resources themselves to increase capacity.
Such clients may use cloud resources as an extension of their existing HPC for use with all workloads, or segment and only use cloud for adhoc non-critical (and loosely coupled workloads), or perhaps just for ‘bursting’ into the cloud to deal with peaks in demand either because the planned workload exceeded expectations and bursting was needed to complete it on time (e.g., CGI rendering), or bursting was employed to speed-up execution and produce simulation results more quickly. By using the cloud as an adjunct enables these companies to extend the usefulness of their existing on-prem systems, and any new systems they deploy can be designed with less peak performance capacity by being able to burst into the cloud whenever needed.
Given that the corporate will already have on-prem and/or private cloud infrastructure, cloud HPC tools will be needed that can interface with the existing 3rd party workload schedulers. Equally, any cloud HPC resources that are employed may need to be matched to the on-prem resource types already in-use, hence intelligent tooling will be needed that can analyse individual workload requirements and provision the most appropriate cloud resources across the myriad of available instance options from the CSPs, and map the workload accordingly across the on-prem and cloud infrastructure.
Depending on the workload, the corporate may also decide to use spot/preemptible instances to complete batch processing tasks without loading other cloud resources and/or as a way of managing cost.
Cloud-native corporates
Corporates in sectors such as financial services, retail, media, gaming, manufacturing and logistics that are dependent on high-performance compute to drive their deep learning models, simulations and business decisioning to maintain a competitive edge but with insufficient funds and/or interest in deploying and managing dedicated HPC resources on-prem hence reliant on such resources being provided via the cloud.
Given the mission-critical nature of their workloads, such corporates are likely to follow a multi-cloud strategy to provide resiliency and de-risk dependency on a single provider. Selection of resources may also be driven by corporate sustainability goals, with a preference for CSPs and/or specific CSP data centres that maximise use of renewables.
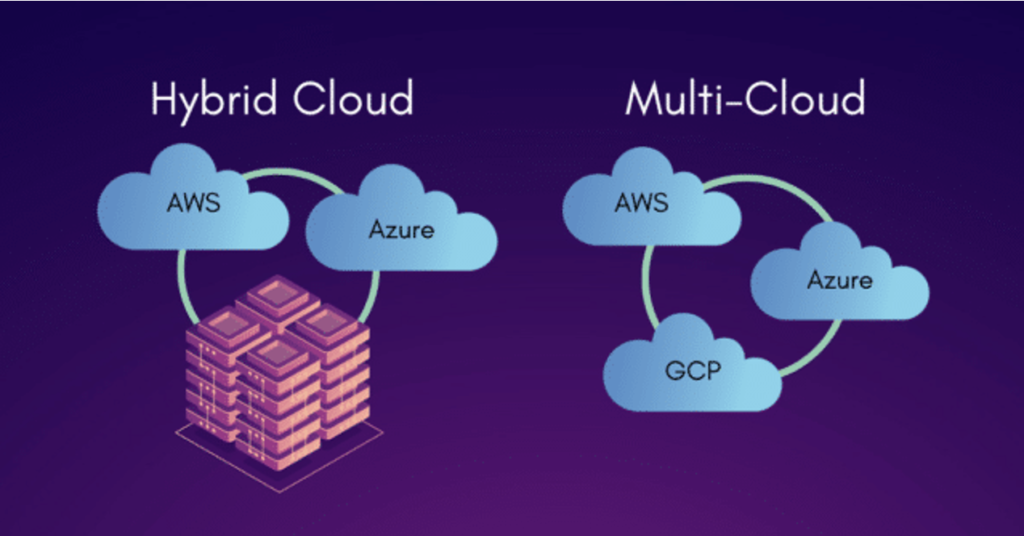
Intelligent tooling will also be needed for use by the corporate in parallelising their workloads and integrating into their existing DevOps processes, and a dashboard providing oversight of HPC resources employed and workload status.
Startups/scale-ups
Similar to the cloud-native corporates, many startups/scale-ups utilising deep learning for NLP, computer vision etc. are keen on gaining access to HPC resources to accelerate their product development and time to market, and/or would like to develop products and services that can scale up and down in the cloud, but may not have the budget or expertise to achieve this.
Such companies are therefore wholly dependent on automated tools that enable them to programmatically control their usage via DevOps interfaces and dynamically switch between different CSPs and instances to minimise their costs. Primary usage will be via preemptible resources, and startups may also choose to use older generation instances to meet budgetary constraints.
Introducing YellowDog
YellowDog is a pioneer in the cloud HPC space, providing solutions that enable intelligent orchestration, scheduling and provisioning at scale across on-prem, hybrid and multi-cloud environments and delivering on all the requirements outlined above.
In addition to providing benefits to companies already employing HPC, they’re unique in being able to generate clusters delivering HPC levels of compute using spot/preemptible instances hence are well placed to support the new breed of companies needing access to HPC performance levels at an affordable price and to provide startups with a base platform that enables them to easily develop a new autoscaling product or service hence reducing their time to market and simplifying development.
A particular speciality of YellowDog is the ability to rapidly spin-up massive scale HPC clusters that aggregate resources from multiple CSPs and/or across multiple regions to circumvent the scaling limits in any particular CSP; in 2021, YellowDog successfully demonstrated creation of a cluster utilising 3.2million vCPUs on AWS to run an HPC workload with 95% utilisation, and achieved this feat in under an hour.
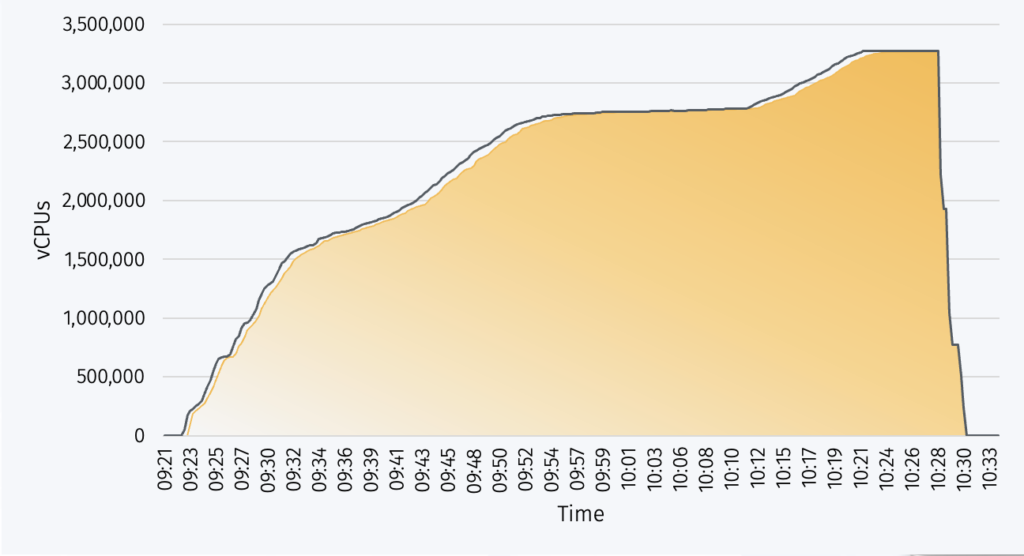
Figure 4 Scale-up to 3.2 million vCPUs and rapid scale-down on job completion (YellowDog; AWS)
The YellowDog platform provides a straightforward GUI enabling engineers and scientists to use the platform without needing to be HPC specialists, and also provides a sophisticated dashboard and API access for managing workloads and provisioning preferences, including an ML-based prediction of completion time thereby enabling customers to easily flex the resources being employed to meet a particular deadline or budgetary constraint.
Unique in the market, YellowDog also compiles a realtime insight on the myriad of different instances offered by the main CSPs[1] with regard to their machine performance, pricing, and use of renewables, and utilises this intelligence within the YellowDog platform to deliver optimal provisioning for its clients.
Whilst there are other companies offering solutions to help clients with their cloud orchestration and management, only YellowDog provide orchestration twinned with intelligent scheduling and provisioning at sufficient scale to deliver compute capabilities at HPC performance levels, and at a price point using spot/preemptible resources that meets the growing industry demand, and via a platform and set of tools that enable all to enjoy the benefits of cloud HPC.
Takeaways
The world is speeding up.
Easy access to HPC levels of compute via the cloud is changing the economics of product development, increasing the pace of innovation and enabling corporates to increase agility, accuracy, and critical insights in today’s data-driven economy. By harnessing preemptible instances and spot pricing, even the smallest of companies and startups can now afford to run HPC workloads.
Preemptible instances ensure that cloud resources do not lie idle, and bring environmental benefits as well as incremental revenues for the CSPs and lower costs for companies utilising the cloud – a veritable win:win for all, and demonstrates that HPC systems in the cloud can be cost-comparable to on-prem alternatives whilst bringing many advantages.
Harnessing the potential of cloud HPC whilst meeting all other business objectives though is no mean feat and will be dependent on intelligent tooling. YellowDog is a pioneer in this space and a perfect partner for any business looking to leverage cloud HPC resources to gain a competitive edge.
[1] Amazon Web Services (AWS), Google Cloud Platform, Microsoft Azure, Oracle Cloud Infrastructure and Alibaba
Cybersecurity innovation critical in combatting the inexorable rise in cyber threats and ransomware attacks.
Bloc invests in technology areas that underpin the future growth and prosperity of the digital age. Cybersecurity, and in particular the challenges companies face as they move operations online and into the cloud, is a growing area of importance and innovation.
The landscape for security teams is rapidly changing. Digital transformation, accelerated by Covid and remote working, is driving a rapid uptake in cloud utilisation.
Hybrid multi-cloud & remote working practises are dramatically expanding the attack surface as workforces access company IT systems from unsecured devices (home PCs, tablets) and over unsecured WLANs (home, coffee shops) thereby tearing down the single security perimeter that security teams have previously come to rely upon.
Competitive pressures driven by DevOps & CI/CD working practises are leading to mistakes in cloud configuration and deployment of unauthorised shadow IT, both of which are creating additional vulnerabilities within company networks – Verizon estimates that 82% of enterprise breaches should have been stopped by existing security controls but weren’t, and 79% of observed exposures were in the cloud compared with 21% for on-premise assets.
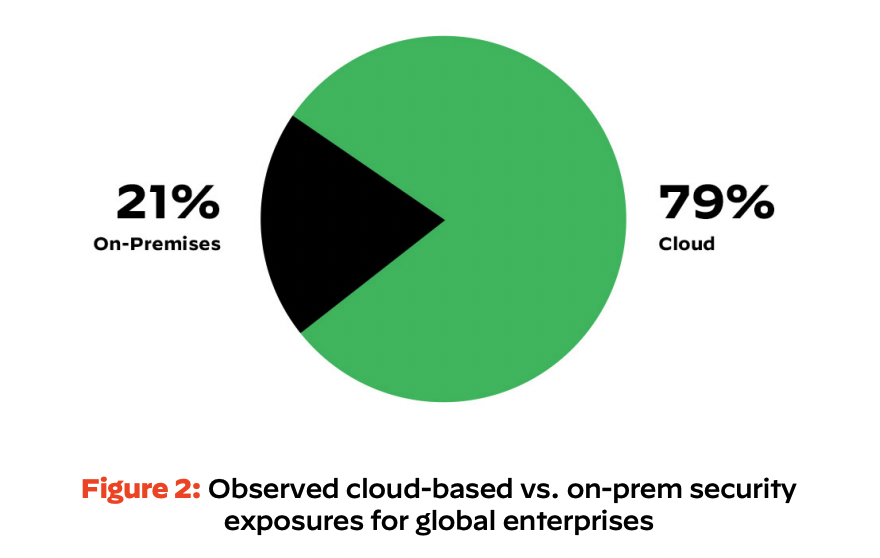
Worst still, zero-day vulnerabilities introduced or exploited within the systems and software of companies’ suppliers is on the rise – a Trojan horse in effect that a business has very little control over, although startups such as Darkbeam are seeking to help companies manage the risk.
Cyber-attacks and the resultant data breaches are expensive, erode customer trust, damage brand reputation and can ultimately stop a company in its tracks.
And yet despite their efforts, many companies are being overwhelmed by the magnitude of threats they face, and are ill-equipped to differentiate between real threats and false alerts coming from their networks.
Survival will be dependent on the development of intelligent tools leveraging advanced AI/ML that can augment and support security teams in their ever-lasting battle with the cybercriminals.
Key areas for innovation identified by Bloc
We have identified a number of cybersecurity areas for innovation:
- Use of few-shot learning AI techniques for detecting zero-day exploits with unknown signatures such as those introduced through supply chain attacks
- Supply chain attacks are massively on the rise (650% annual growth) and involve cybercriminals inserting malicious code into ISV (Independent Software Vendor) products to gain access to companies downstream as was the case in the SolarWinds attack in 2020
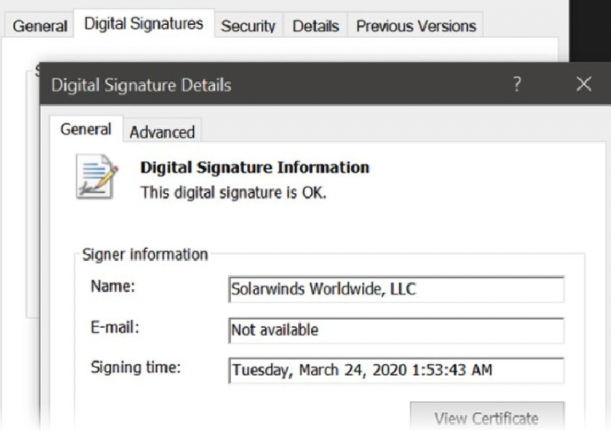
- Methods for obfuscating existing networks to inhibit attackers without the company needing to re-architect
- Enclave Networks is one such company helping its clients to ‘darken’ their networks through the introduction of invisible network access gates
- Implementing zero-trust principles to prevent attackers from moving laterally through the network after gaining access via infected systems
- Zero-trust assumes that everyone in the network could be a bad actor, hence all activity is continuously monitored for behavioural anomalies and access to individual systems managed via granular privileges and more robust authentication methods
- Introduction of cyber deception platforms and honeytraps that lure attackers into revealing themselves thereby enabling security teams to shut them down before they cause any serious damage
- CounterCraft, for example, provide a cyber deception and counterintelligence platform designed to detect intrusion and insider threats before attacks are perpetrated
- Supporting anomaly detection at scale, especially for Industrial IoT networks comprising huge numbers of devices
- Realtime anomaly detection becomes especially challenging in the IoT space as the number of devices scale into the millions. One way to tackle this (pioneered by Shield-IoT based on work conducted within MIT) is to compress the network and resulting data into a smaller coreset enabling context-free highly accurate anomaly detection in minutes instead of hours or days
The market opportunity is clear
Cybersecurity software & tools in 2020 was worth $12 billion in the UK, $26.5 billion in Europe and $78 billion globally and is projected to grow to $118 billion globally by 2024. The cybersecurity market for hardware & software combined is expected to exceed $200 billion by 2024 and reach $372 billion globally by 2028.
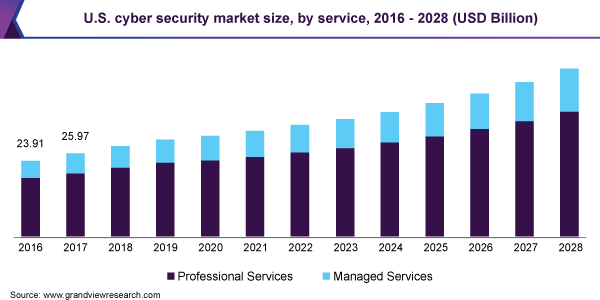
Managing cloud vulnerabilities is a race between attacker and defender and therefore ripe for new entrants bringing fresh ideas and utilising the latest technology to deliver anomaly detection, behavioural profiling and automated tools for supporting security teams and those companies wanting to take their business operations into the cloud.
Get in touch with Bloc
We’re always looking for entrepreneurs innovating across cloud, connectivity, data science and security. Get in touch with our investment team below.